Maretha Prinsloo and Paul Barrett
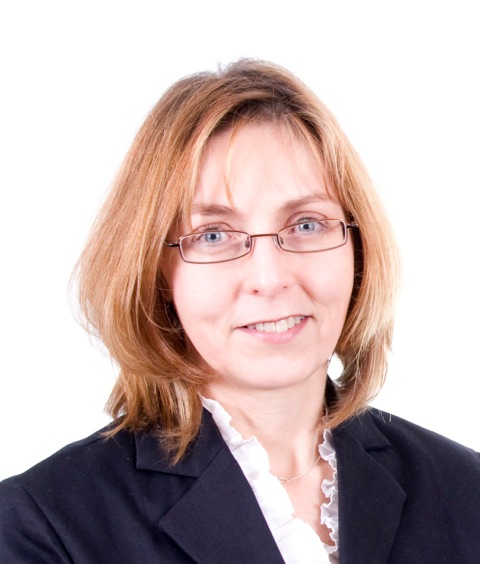
Maretha Prinsloo
Abstract
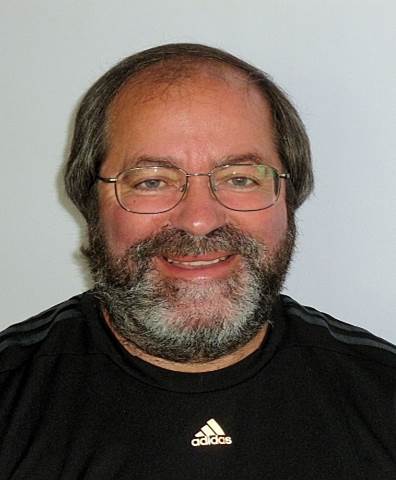
Paul Barrett
Given the spectrum of consciousness as postulated by various consciousness theorists, cognition, according to Wilber’s All Quadrants All Levels (AQAL) metatheory merely represents a developmental “line” or “stream”, and does not encapsulate the essence or apex of consciousness. However, as proposed in a previous article on consciousness theory (Prinsloo, 2012), the fractal nature of the various “streams or lines” of development reflects that of the overall evolutionary emergence of consciousness, all of which involve processes of increasing differentiation followed by increasing integration of subcomponents.
This article focuses on cognition, which is of critical importance within educational and work environments, as well as within the context of leadership assessment and development. Up to a point, cognitive factors enable the emergence of consciousness, and very importantly, the implementation of one’s world view, or level of awareness, as covered in a previous article in this journal. This does not imply a linear relationship between cognition and consciousness. People with high levels of cognitive capability, for example, can be found at any of the various levels of consciousness as hypothesised by various consciousness theorists and developmental psychologists (Prinsloo, 2012). Here, cognition is also not merely regarded as intellectual “ability”, which has been the dominant perspective within psychology and psychometrics for more than a century.
The view proposed here involves an integration of various scientific questions posed by different research traditions within the field of intelligence and cognition, aimed at addressing the:
- “what” of intelligence as embraced by Differential psychology and the IQ tradition.
- “how” of thinking as reflected by the Information Processing paradigm, and cognitive and computational neuroscience;
- “when” of cognitive capacity explored by Developmental psychologists such as Piaget and Vygotsky; and the…
- “where” of competence as researched by the Contextualist school
While focusing on a theoretical model of cognitive processes and a methodological approach for the measurement of cognitive capabilities and preferences, as well as contextualising cognition within the real world and the broader spectrum of consciousness.
Cognition and Intelligence
Few terms in Psychology have elicited the amount of controversy that the concept of intelligence has (Whiteley, 1977). Since the 1921 symposium convened by the editors of the Journal of Educational Psychology, asking for definitions of intelligence from 14 expert contributors, there have been several later attempts to develop a definition of intelligence. For example, in 1986, Robert Sternberg and Douglas Detterman published the definitions from 24 of the leading researchers in the area, who were asked the same questions as were asked of the 14 experts in 1921. Again, as in 1921, disagreement was common. In 1996, Ulric Neisser and 8 colleagues from the APA Task Force on Intelligence published what was meant to be a definitive set of statements on intelligence, in an article entitled: “Intelligence: Knowns and Unknowns”. In 2012, Richard Nisbett and colleagues updated the APA Task Force 1996 position statements based upon incorporating new thinking and findings from a further 15 years of research into intelligence. Their article entitled: “Intelligence: New findings and theoretical developments”, used a working definition of intelligence provided by Linda Goffredson (1997):
[Intelligence] . . . involves the ability to reason, plan, solve problems, think abstractly, comprehend complex ideas, learn quickly and learn from experience. It is not merely book learning, a narrow academic skill, or test-taking smarts. Rather it reflects a broader and deeper capability for comprehending our surroundings— “catching on,” “making sense” of things, or “figuring out” what to do (p. 13).
Intelligence is typically defined in terms of concepts such as learning, problem solving, memory, executive control, judgment, speed and the ability to abstract. In reviewing all these conceptions, it appears that the notion of intelligence seems quite arbitrary – or rather – the research community has failed to agree on a definition for the concept. Despite their obvious limitations, some of these definitions seem to have gained general acceptance mainly because of their parsimony and intuitive appeal. Intelligence is therefore a problematic concept in that it is of a fuzzy nature incorporating many important features for which no definite criteria can be determined.
Not only attempts to define intelligence, but the concept per se, have repeatedly been criticized. Some theorists regard the term intelligence as useful in everyday language but do not view it as constituting an adequate scientific concept, even though it is regarded as scientifically useful by others. Maraun (1998) perhaps put this most eloquently:
The relative lack of success of measurement in the social sciences as compared to the physical sciences is attributable to their sharply different conceptual foundations. In particular, the physical sciences rest on a bedrock of technical concepts, whilst psychology rests on a web of common-or-garden psychological concepts. These concepts have notoriously complicated grammars [of meaning]” (p. 436).
A technical concept is a concept defined by a specialized or expert community, and employed within a narrow, technical field of application. A common-or-garden concept, on the other hand, is a concept with a common employment in everyday life (Baker & Hacker, 1982). Common-or- garden concepts are taught, learned and understood by the person on the street, and have meanings that are manifest in broad, normative linguistic practices (p. 453).
These psychological constructs that Maraun refers to as “common-or-garden” concepts, such as “depression” “intelligence”, “dominance”, “happiness” and “fear”, are of interest to laymen and specialists alike who all have a greater or lesser degree of understanding of the meanings involved – the latter of which is largely rooted in grammar/semantics. Ter Hark (1990), amongst others, also points out that there are no rules available for the measurement of these psychological concepts as they are not embedded in normative practices of measurement.
Scientifically capturing the essence of these complex, intangible and largely descriptive concepts is one of the core challenges in psychological research and has resulted in a polarisation of approaches ranging from quantitative to qualitative observation and interpretation as well as speculation.
As early as 1965, Guttman foresaw and attempted to resolve the controversy surrounding the value of the concept of intelligence by suggesting that one should refer to the degree of intelligence of an act rather than to the concept as such. Michell (2013) has recently expanded on this position in his article entitled “Constructs, inferences, and mental measurement”:
“Psychologists named constructs using familiar mental terms, such as “anxiety”, without dwelling upon the construct’s meaning, thinking it sufficient that constructs were “operationalized” via test scores. A construct’s “nomological network” became an issue of operational “definitions”, often involving scores on a range of tests as, for example, general intelligence is thought of as “operationally defined” via factor analysis of scores on several tests (Flynn, 2007). Thought of this way, constructs are really dispositional concepts, that is, “defined” in terms of their putative effects, but, as has already been stressed, effects cannot satisfactorily define constructs. For example, general intelligence is sometimes “defined” as abstract problem-solving ability, which says nothing about the character of intelligence per se, but merely specifies it as the otherwise unknown cause of successful abstract problem-solving behaviour. Furthermore, the construct’s dispositional label (e.g., “abstract reasoning ability”), because it seems to admit of degrees of more and less, is taken, fallaciously (Michell, 2009), to vouchsafe the construct’s presumed quantitative structure (p. 16).
Given the above, it is not surprising that so many have ‘constructed’ definitions of intelligence that differ significantly in some cases from one another. From these more thoughtful philosophical perspectives, the rush to create ‘models for intelligence’ was always doomed to failure. The recent article by Ken Richardson (2013), incorporating new evidence from molecular biology and systems theory dynamics adds to a growing wisdom on these matters. However, it is important for our purposes to briefly outline how models for intelligence (as cognition) evolved; because it is this history that provided the catalyst for the expanded approach to cognition that resulted in the first author’s theoretical propositions and assessment design.
A Brief History of Models of Intelligence
Galton’s Mental Ability
Sir Francis Galton was perhaps the first scientist to approach the systematic study of individual differences in cognition within humans; his approach eventually became referred to as ‘differential psychology’. His aim was to classify, measure, and explain the variety of phenomena which were associated with behaviours and cognitive performance in humans. In his book, Inquiries into Human Faculty and Its Development, Galton (1883) set out a variety of tests and assessment methodologies for measuring basic human capabilities; these mostly consisted of sensory discrimination tasks and speed of response (reaction times and suchlike). Although he used the phrase ‘mental ability’ to indicate that which he desired to measure, he ultimately failed to construct a ‘measure’ of what he himself, and many others, accepted as ‘intelligence’.
Binet’s Scholastic Assessments
In 1905, responding to the need to assess children’s learning and intellectual performance within the Paris school system, Alfred Binet created what has since been promoted as the very first ‘intelligence’ test (Jensen, 1998). This was comprised of many kinds of task, some borrowed from Galton’s earlier work like the discrimination of weights, but the majority encompassing more cognitively complex assessments of reasoning, judgment, and verbal comprehension. But, in contrast to the ‘received wisdom’ promoted by many psychologists, Michell (2012) has noted that Binet did not claim his test measured “intelligence”:
“This scale properly speaking does not permit the measure of the intelligence, because intellectual qualities are not superposable, and therefore cannot be measured as linear surfaces are measured, but are on the contrary, a classification, a hierarchy among diverse intelligences; and for the necessities of practice this classification is equivalent to a measure” (Binet & Simon, 1980, pp. 40–41).
Psychometrics and Multiple Ability Models
Also referred to as the structural or psychometric approach and dominant during the first half of the twentieth century, this approach focuses on individual differences in order to reveal the structure of the intellect in terms of content-related abilities. It largely consisted of efforts to conceptualise intelligence by means of factor analysis (Wagner, 1987; Wagner & Sternberg, 1984). In this approach common sources of variance among individuals are researched as unitary psychological factors or attributes. The identification of “general” versus “specific” intelligence became a major issue at this point. The contributions of British theorists Spearman and Burt, and the American theorist Cattell, are relevant here. Spearman introduced the method of factor analysis, thereby laying the foundation of Differential psychology. In his later work he produced his famous two-factor theory of intelligence. This represents intelligence as comprising both general ability, designated as “g” and specific abilities, or “s”. The general factor pervades the entire range of intellectual performance, while the specific factors are relevant only to specific knowledge domains. This approach however, reflected a focus on “content”, or the “what” as opposed to “process” or the “how” of thinking. Spearman’s work has been criticised for not always being supported by the data, and the sampling of only a small part of human cognitive capability (that which is related to scholastic achievement in particular).
In an attempt to overcome the shortcomings of existing theories at that stage, Burt integrated various theoretical positions (as discussed already) and in 1949 developed a model of the structure of mind: a hierarchical exposition of mental structure. This hierarchy also consists of “specific factors” at the lowest level, which are integrated into broader categories, or “minor group factors”, which are integrated into fewer “major group factors”, which are all aspects of “g” (Sternberg, 1977a). Some of Burt’s work has been discredited on the grounds that it is unsuitable for serious scientific consideration. It has nevertheless proved to be a rich source of hypotheses for subsequent research. Vernon formulated an alternative hierarchical model at four levels of abstraction. He proposed the following hierarchy: general ability at the top, followed by: verbal educational and spatial mechanical abilities at the second level, followed by a third level of minor group factors and a fourth level of specific factors.
In the United States, Thurstone developed the statistical technique of multiple factor analysis. By doing so he directed worldwide attention to the developments in American Psychology. His theoretical position was in opposition to that of the behaviourists in that he ascribed cognitive behaviour to occurrences within the individual, rather than seeing it merely as a response to external stimuli. He also criticised the basis on which items for IQ tests were selected, and disregarded the mental age concept. Thurstone allowed empirical findings to guide the development of his theory, thereby maintaining an empiricist position. His method revealed seven primary mental abilities, namely: spatial ability (s), perceptual speed (p), number facility (n), verbal meaning (v), rote memory (m), word fluency (w) and inductive reasoning (i). According to Thurstone, general factors exist only by virtue of the correlations between primary abilities. Thurstone’s theory initially seemed to completely discredit the British theory of “g”. Cattell however, suggested in 1941 that “g” might be obtained as a second order factor among Thurstone’s primary abilities (Snow, Kyllonen, & Marshalek, 1984; Sternberg, 1977c; Verster, 1982).
Guilford’s Structure-of-Intellect (S-I) model was proposed in an attempt to integrate the proliferation of ability factors in intelligence research stimulated by Thurstone’s work. According to Guilford, abilities can be represented as a three-dimensional cube, the dimensions being:
- “operations” (evaluation, convergent thinking, divergent thinking, memory, cognition);
- “products” (units, classes, relations, systems, transformations, implications); and
- “contents” (figural, symbolic, semantic, behavioural).
The result is a three-way matrix of 120 cells, each made up of a unique combination of an operation, content and product, and each representing a unique mental ability. The proposal of such a large number of abilities as independent factors has, however, been viewed sceptically, especially since most empirical findings contradict it. Although the principles of classification identified by Guilford provide a logical and convenient frame of reference, they can also be criticised for being largely arbitrary. His model has, however, transcended the narrow “content” focus of the Differential approach, and has served as a rich source for ensuing research. The S-I model also provided a basis for the identification of various levels of complexity at which individuals prefer to process information, and thus underlies a number of complexity oriented systemic models. Guilford’s contribution in this regard has, however, not really been referenced by systems theorists whose work closely resembles it (Snow et al, 1984, Sternberg, 1977c; Verster, 1982; Wagner & Sternberg, 1984).
There have been many other attempts at creating taxonomic systems for the representation of cognitive abilities (Prinsloo, 1992). Some of these models are more useful than others.
According to Cattell, as well as Horn’s later Ïfluid-crystallisedÓ theory, a generalised factor can be subdivided into a fluid ability (Gf) and a factor of crystallised ability (Gc). Gc is primarily applicable to verbal-conceptual tasks, whereas Gf to genetic and neurological determinants as well as factors of incidental learning. Whereas verbal comprehension and the cognition of semantic relations load highly on Gc, induction, general reasoning and the cognition of figural relations load on Gf. An impressive body of evidence supports Cattell’s theory which with time he revised and extended. His choice of item content to evaluate Gf can, however, seriously be questioned – especially within cross-cultural and cross-gender milieus (Gustafsson, 1988; Sternberg, 1981; Verster, 1982).
Horn also modified his theory and in a 1986 model, analysed ability within an information processing hierarchy with levels of sensory perception, associational processing, perceptual organisation and relational eduction (Gustafsson, 1988; Sternberg, 1981).
Guttman proposed a “facet theory” in which the concept facet refers to two sets of elements: set A and set B. If set A consists of all the verbs in a language, set B consists of all the nouns. The Cartesian space AB would include all possible ab relations. Set A and set B would then be facets of space AB. A Cartesian space can, however, have any number of elements. With this model, Guttman (1965; Sternberg, 1981) thus synthesised two distinct notions into a single theory. The notions include (a) differences in the type of task material (the domain) and (b) differences in the degree of task demand (the range), which ranges from an analytical core (nature of the type of relations to be cognised) to achievement (the application of rules). A radex can thus be seen as a doubly ordered system which combines a simplex (a set of variables representing an order of complexity) and circumplex (variables differing in the type of ability they define). Guttman applied a form of non-metric scaling in the development of his radex model. It does therefore not derive solely from factor analytic research. He also made use of rank order data in studying interrelations among intelligence scores.
The hierarchical models that are based on correlational data and reflect content specific abilities can, however, be regarded as constituting the most widely held view within the Differential paradigm.
Although the Differential approach stimulated enthusiasm in research for most of the previous century and has led to the accumulation of a wealth of research findings, it can be criticised on a number of grounds (Carroll, 1974; Gustafsson, 1988; Horn, 1986; Howe, 1988; Sternberg 1977c; 1983), including:
- The primary function of the differential approach was phenomena detection, not explanation (Haig, 2005). It is a paradigm for describing aggregate differences between groups of individuals. While this is an essential component of any investigative science (phenomena detection), in essence, it is limited to description, not explanation, with a focus on the development of selection instruments rather than an attempt to explain the phenomenon of intelligence;
- the hierarchical model of ‘g’ as a general factor of intelligence is a description of a phenomenon which has practical value, but again, lacks coherent causal explanatory theory. It can be ‘constructed’ from analysis of covariance between many kinds of ability tests, or from any set of independent components which share multiple underlying causal constituents (Thomson’s ‘bonds’ model; 1916, Bartholomew, Deary, and Lawn, 2009), or from a dynamical systems model (van der Maas et al, 2006);the cultural bias of almost all ability tests;
- Although intercorrelations of ability scores are mostly positive, different sources of variance are often found. Contrary to a widely held belief, the speed and power of thinking represent two different constructs that are not necessarily linearly related;
- The Differential approach failed to consider the nature of thinking processes and merely focused on the person’s current “ability” to apply convergent reasoning (logical-analytical) processes to deal with highly structured problems in a specific content domain – thereby focusing on a highly selected and limited aspect of cognition.
The concept intelligence is thus of a descriptive rather than an explanatory nature. It is useful for labeling and predictive purposes. However, stating that someone performs well because he or she is intelligent is no more meaningful than saying that someone produces much because s/he productive. The well-known cognitive theorists, Sternberg and Feuerstein, have taken note of the more contemporary position and provide generic and qualifying descriptions of the concept of intelligence.
The position taken on the concept of intelligence in this paper is that:
- the concept is useful for practical purposes;
- it is used in a descriptive rather than explanatory sense;
- no attempt is made to construct a “correct” definition;
- the construct represents a prototype;
- the concept of intelligence primarily refers to the quality of conceptualisation processes, or the way in which a person interprets the world meaningfully (Prinsloo, 1992).
It is proposed here that this emphasis on conceptualisation can be fleshed out in terms of both structural and processing aspects. In terms of the cognitive structures involved, the degree of intelligence of a mental act may be associated with:
- the number and diversity of structures;
- structural representation at different levels;
- the potential size and complexity of the structures;
- the number and quality of inter-structural links, or the degree of integration;
- the completeness and clarity of structures;
- the modifiability, or potential adaptability of structures;
- the potential for resistance to structural interference;
- the absence of blocking / resistance to the formation of information structures;
- the strength of the tendency toward increasing order, integration and differentiation (Prinsloo, 1992).
The degree of intelligence of a cognitive act can also be related to the following functional aspects of information processing:
- the speed of processing;its goal directedness;
- the flexibility and fluency of thinking processes;
- the degree to which feedback is integrated to result in learning;
- processing power, intensity, energy and momentum;
- processing activity on all the identified levels (the levels referred to in the proposed theoretical model discussed later in this article, namely the performance, metacognitive, general or rule and subconscious levels);
- the interaction and integration of processing activities between the different levels of processing, including intuitive awareness of subconscious links; and
- the degree of automatisation of processing procedures (Prinsloo, 1992).
Cognitive Models and the Information Processing Approach
Contributions from a large number of disciplines during the 1940s and 1950s culminated in the emergence of Cognitive psychology in the mid-1960s (Dellarosa, 1988). This discipline formed part of the larger field of cognitive science which includes a wide range of subdisciplines, such as philosophy, linguistics, psycholinguistics, computer science and neuroscience, all of which focus on higher mental processes. This new approach enabled the researcher to investigate internal states and processes in a scientifically rigorous manner. It did so by focusing attention on limited cognitive tasks to facilitate the collection of data on cognitive processes.
Cognitive Psychology encompasses subdisciplines such as the information processing approach (as part of Experimental psychology), the Artificial Intelligence (AI) perspective and Neuroscience. The cognitive revolution brought an end to the Behaviourist monopoly in psychological research.
Experimental psychology (Royce & Powell, 1983) is associated with a focus on cognitive processing and encompasses the information processing approach. A variety of disciplines also contributed to the development of the information processing perspective on intelligence. These include ideas from Symbolic logic and Cybernetics on the one hand, and the Wurzburg and Gestalt schools on the other. Logic and Cybernetics contributed the idea of symbol manipulation systems. The latter involves the rigorous deduction of ideas which are represented by symbols. The Gestalt school, in turn, yielded ideas on the organization of associations and selective goal-oriented searches (Newell & Simon, 1972; Simon, 1979).
The “information processing” metaphor, however, has its origin in functionalism, which lies within the Behaviourist orientation, as well as in the computing and informational sciences (Royce & Powell, 1983). The stimulus-response concept of Behaviourism is seen as a main contributor to the development of this approach (Sternberg, 1977c). Although the Information processing approach partly developed from Behaviourism, a shift occurred from the examination of observable phenomena to the study of the unobservable.
Political, social and technological developments further impacted on the emergence and expansion of the Information processing paradigm. The inadequacies of the Psychometric approach, in particular, resulted in disillusionment and an increasing shift away from traditional psychometrics in the 1960’s, to a stronger research focus on the Information Processing paradigm.
With the appearance of the electronic digital computer, the brain-computer metaphor was soon adopted for its heuristic usefulness. In the 1930s certain similarities between neurological organization and computer hardware were noticed. The computer opened up new possibilities and enabled researchers to model cognitive processes to develop artificial intelligence (AI) systems. Initial scepticism that the computer could adequately simulate human cognition had to be overcome. Between 1956 and 1972, well developed theories of intelligence emerged to accommodate this new metaphor. These theories focused mainly on the types of induction involved in concept attainment and sequence extrapolation tasks (Dellarosa, 1988; Newell & Simon, 1972; Simon, 1979).
In 1960 Newell, Shaw and Simon, as well as Miller, Galanter and Pribram, published major works which originated the Information processing approach. They put theories forward that could be implemented and tested by means of the digital computer. Here the elementary information process (PIP) was regarded as the fundamental unit of analysis. Newell and Simon expanded their notion of an elementary information process in a 1972 publication where they introduced the concept of “production systems”.
Warnings about shortcomings inherent to the information processing approach soon followed. A close relationship was, and still is, however maintained between cognitive psychology and Artificial Intelligence.
Whereas psychometric theories differ mainly in terms of the identification of factors and the inter-factorial relationships, information processing theories differ in terms of the “level of processing” focused on, and ranges from reaction time studies on a perceptual-motor level to the level of complex reasoning and problem solving. Both hierarchical and non-hierarchical models have been proposed in this regard (Sternberg, 1983).
Although theories contributing to the information processing approach are often applauded for their precision and testability unrivalled by other accounts, they can be criticized on a number of grounds (Dellarosa, 1988; Horn, 1986; Newell, 1973), including the following:
- Even though some theorists regard the exploration of the underlying mechanisms of Cognitive Psychology as a critical prerequisite for a more holistic understanding of thinking, their constructs lack external validity. This could, however, well lead to the development of an isolated laboratory Psychology that bears no resemblance to everyday cognition.
- It is limited in terms of the type of performance studied because a large proportion of processing research makes use of a small number of units of cognitive behaviour. Such findings cannot be generalized to a wider range of intellectual application.
- The tasks developed to measure cognitive processes are of a specific nature and are only obliquely related to educational and everyday goals. Information processing research can also be criticised for not justifying the grounds on which tasks are selected.
- The rigidity of computer models contrasts sharply with the flexibility of human performance. Gestalt psychologists, for example, believe that understanding, which is a fundamental characteristic of human cognition, cannot be adequately simulated by a computer.
- The main criticism levelled against the experimental approach is that it does not accommodate the role of individual differences in processing.
To summarise, Experimental Psychology incorporates many paradigms, but tends to be dominated by the Information Processing approach. The various Information Processing theories see intelligence in terms of mental representations, the processes underlying these representations and the way in which these processes are combined. Its basic research question is concerned with the way people think. The identification and verification of hypothetical cognitive processes is regarded as a primary research goal. The methodology includes techniques such as content analysis, mathematical modelling, calculations of response time or error data and the computerized simulation of processing. The unit of analysis is the information process. It is fundamental in the sense that on a theoretical level it cannot be broken down into simpler components. This approach aims at providing a means of breaking task performance down into underlying mental processes.
The Integration of the Differential and Information Processing Approaches
The next historical phase to have emerged within the field of intelligence research is the integration of the Differential and the Information Processing approaches. The merging of the correlational and experimental approaches was inevitable considering their theoretical complementarity. Two main schools of thought have been influential in this regard. These are the “Cognitive Correlates” and “Cognitive Components” approaches as primarily published on by Prof. Sternberg from Yale. Both approaches attempt to establish statistical relations between performance on psychometric tests and laboratory tasks.
The methodology of the Cognitive Correlates approach involves the correlation of psychometric and laboratory results, mainly reaction times. Results are then factor analysed to explore common sources of variance. This is thus an attempt to identify information processing skills that predict psychometric scores. In addition, it aims to overcome criticism of the laboratory approach as an oversimplification. Research results however, are not clear and consistently produced (Egan & Gomez, 1985; Guilford, 1967; Larson & Sacuzzo, 1989; Pellegrino & Glaser, 1979; Sternberg, 1979).
Subsequent research had as its aim the analysis of more complex cognitive tasks in terms of their processing requirements. This came to be known as the Cognitive Components approach. This approach is task analytic in nature and attempts to specify the information processing components of tasks used for assessing mental abilities. It involves the theoretical and empirical analysis of task performance on standardised aptitude tests to develop performance models. Methods used range from intuitive analysis to computer simulation, protocol analysis and mathematical modelling, as well as combinations of these methods. The information processing demands of psychometric tasks are thus analysed. This approach is often referred to as S-O-R research in that the emphasis is on the stimulus and the examinee. Tasks used in these analyses include: verbal and geometric analogies, verbal classifications, syllogisms and series completions. The most prominent model that emerged within this methodological orientation is Sternberg’s Componential theory of intelligence (Chipma, Segal, & Glaser, 1985; Embretson, Schneider, & Roth, 1986; Pellegrino & Glaser, 1979; Sternberg, 1977a; 1977c, 1985).
There were also other attempts to integrate the information processing and the differential approaches. Examples are Royce and Powell’s (1983) “Systems Perspective” that focused on structure as a basis for understanding function. They looked at psychological functioning in an integrated and holistic manner focusing on styles, values, cognition, affect, sensory systems and motor systems.
Despite various attempts to consolidate the above theoretical stances, tremendous diversity still exists in the field of Cognitive Psychology. The Contextualist, Developmental, Psycho-physiological and Production (Artificial Intelligence) approaches can be summarised as follows.
The Contextualist Approach
Based on criticism of the Differential and Information Processing paradigms, the Contextualist approach to intelligence research also emerged. Contextual factors have often been downplayed by theorists whose bias towards genetics led them to reduce intelligence to a manifestation of a differentially distributed biological attributes and used academic performance as an indicator in this regard.
Criticism was also widely levelled against the Information Processing approach, specifically research that took cognition completely out of context. Contextualist educators and psychologists introduced the constructs of cultural bias and fairness, did a great deal of research on practical intelligence, and increasingly emphasised contextual factors (Berry, 1974, 1984; Ford & Tisak, 1983; Frederiksen, 1986; Gordon & Terrel, 1981; Keating, 1984; Neisser et al, 1976, 1978; Schlecter & Toglia, 1985; Scribner, 1986).
Examples of the topics researched by Contextualists are:
- the application of ethnographic and experimental techniques to study work performance;
- expert-novice comparisons of job-related skills;
- the investigation of non-academic intelligence and everyday problem solving; and
- cross-cultural factors.
The Developmental Perspective
An interest in a Developmental perspective on intelligence has prevailed throughout the above mentioned phases and has culminated in different theoretical positions (Broughton, 1981; Gallagher & Reid, 1981; Keating, 1984; Sigel & Cocking, 1977). These include emphases on Developmental Structuralism, Genetic Psychology and Genetic Developmental Epistemology. Major theoretical contributions in Developmental Psychology were made by theorists such as Piaget and Vygotsky.
Piaget took a developmental approach to the relationship between knowledge and reality. He made use of qualitative methods in studying both the underlying structure and the manifestation of intelligence as it develops through qualitatively distinct stages which are triggered by adaptive action.
According to Piaget, cognitive operations are interlinked by means of cognitive structures. A “structure” describes the relationship among mental actions, while operations are the units of logical thinking. He saw cognitive structures as objectively real, organizing features of thought and not merely as theoretical abstractions. Piaget’s theory can be regarded as constructivist in that knowledge results from a progressive “building” or structuring process: the individual adapts to his/her environment by actively organizing information through assimilation and accommodation to create a knowledge base. The functions of assimilation and accommodation are complex elaborations of the process of successive imbalance and re-equilibration.
According to Piaget the structuring of information can, however, be limited by the cognitive developmental phase involved. Development depends on four critical organizing factors, namely biological maturation, experience, the influence of the social environment and equilibration or regulatory processes. Piaget proposed that coherent logical structures underlie thought, and identified four qualitatively different developmental phases in terms of their internal structural organization. These are:
- the sensori-motor stage (birth to approximately two years of age);
- the stage of concrete operations (plus minus two to twelve years of age – often subdivided into a pre-operational and concrete operational phase); and
- the formal operational stage (from twelve years of age throughout adulthood).
Piaget’s contributions mainly provided illustrative and confirmatory evidence without validating findings. He also failed to adequately address the relationship between competence and performance. According to Piaget the concept of “structure” can be used in an explanatory manner in that deep structures explain surface appearances. This view is, however, not characteristic of Structural Functionalism, where the term “structure” refers to surface phenomena, while the term function is used in an explanatory sense. Some thus regard Piaget’s integration of structuralism and functionalism as problematic.
Vygotsky, another prominent developmental psychologist, criticised Piagetian theory for its emphasis on the individual’s construction of internal mental structures without recognition of the important role of social interaction. He also rejected the traditional emphasis on genetics and biological maturation as key contributors having potential “ceiling effects” in intellectual development, and proposed the concept of “learning potential” and “mediation” amongst others.
Vygotsky saw, as a key factor in the development of intelligence, the individual’s internalisation of social interactions. Because Vygotsky includes both developmental and contextual considerations in his approach, his work is regarded as useful and promising for the study of the nature and development of intellectual functioning.
Alongside those who concentrated on the development of broad philosophical and theoretical positions on cognitive development, such as Piaget and Vygotsky, are those researchers who have consolidated the large selection of research findings into an inclusive theoretical model. This was, for example, attempted by Horn (1970, 1978), who reviewed the literature on cognitive development over the entire life span. He found the data to be inconsistent and contradictory, but drew the following broad conclusions:
- The first two years of life seem to be characterized by the development of sensori-motor alertness.
- Development is dependent on the principles of classical conditioning and repetition.
- Around the second year of life, a developmental phase begins which continues throughout the life-span, but is most marked until the sixth year. Piaget identified this as a pre-conceptual phase. In this stage the focus is primarily on perceptual processes used in exploring the environment. Objects are represented symbolically and gradually these impressions are integrated into complex symbol systems. Language development is a related aspect which also takes place during this period.
- Clearly marked changes in cognitive structure occur during the childhood years. From about the ninth to the fifteenth year a shift occurs from the acquisition of basic concepts and symbol systems to the internalization of culture and the development of sophisticated cognitive skills. This period is characterized by the development of crystallized abilities.
- Cognitive development during the adult years has not been researched as thoroughly as that during childhood and youth. Crucial questions addressed in the study of adult cognitive development concern the structure of the intellect and the level of intelligence. Researchers have tried to establish whether cognitive structures remain stable during adulthood; whether a differentiation of preceding phases continues into adulthood and old age; or whether structural de-differentiation occurs.
- With regard to the level of intelligence, there have been attempts to establish if it continues to increase, stabilize or decline during adulthood. The evidence seems to support that of a process of structural de-differentiation.
The Developmental approach has stimulated a wealth of research, and some attempts to integrate this research on a theoretical level as well as to develop elaborate theoretical and philosophical positions. A more detailed discussion of this topic is, however, outside the scope of this article.
Early Psychophysiological Models of Intelligence
A number of speculative psychophysiologically-oriented models also emerged (Eysenck, 1986; Hendrickson and Hendrickson, 1980; Hurlbert & Poggio, 1985; Keating, 1984; Ratcliff, 1981; Simon, 1979; Verster, 1982; Vogel & Broverman, 1964). This field includes a variety of approaches based on philosophies of the mind-brain relationship and range from anatomical studies such as brain damage research and attempts to measure intelligence by means of parameters extracted from spectral decomposition of resting EEGs (electroencephalograms) or AEP (average evoked potentials), to computerized simulations of neurological processes. Most of this early work focused on biological correlates of IQ scores, with explanations couched in terms of speculative concepts of Neural Efficiency (Ertl & Schafer, 1969) and biochemical/physiological features such as myelin sheath density (Miller, 1994) and grey to white matter relative densities (Raz, Millman, and Sarple, 1990; Gur, Turetsky, Matsui, Yan, Bilker, Hughett, & Gur, 1999).
Cartesian Dualism and Structural Monism
A fundamental philosophical position underlying theoretical controversies in cognitive theory, is that of the mind-body relationship. This issue was initially highlighted by the work of Descartes and Hobbes. Descartes regarded the mind as a metaphysical entity in interaction with the material body, whereas Hobbes sees thought in purely mechanistic terms (Dellarosa, 1988). The mind-brain relationship has since been interpreted from various perspectives, including Identity, Dualism, Interactionism, Materialism, Physicalism, Mentalism (Pribram, 1986), Associationist and Evolutionist (Verster, 1982) positions. Pribram’s (1986) work on the so-called mind-brain dichotomy warrants special attention. Pribram integrated various positions on the matter with his explanation of Structural Monism. He interprets mental, or metaphysical, versus physical phenomena, as different manifestations of the same underlying structure, and therefore reflective of the observer’s perspective.
Current Neuroscience and Computational Models of Intelligence
With the introduction of new imaging technologies for in-vivo brain morphology and function during the past 15 years or so, and with increased understanding of brain connectivity, coupled with advances in computational models of cognitive processes and dynamical non-linear systems theory, there has been a revolution in terms of seeking causal explanations of human intelligence and individual differences. The older speculative model of neural efficiency was substantially reworked as neural network organizational adaptability/plasticity. This perspective was formally proposed as a model accompanied with empirical evaluation, which although not entirely successful the hypothesis of neural adaptability and biological self-organization remains tenable (Garlick, 2002; Castellanos, Leyva, Buld˙, Bajo, Pa˙l, Cuesta, Ordez, Pascua, Boccaletti, Maest˙, & del-Pozo, 2011). At the same time as Garlick’s model was being published, so was another influential book detailing evidence of biological self-organizing systems (Camazine, Deneubourg, Franks, Sneyd, Theraulaz, & Bonabeau, 2001). This property of biological systems and its implications for evolutionary models of ‘fitness’ of intelligence was further elaborated upon by Edelmann and Denton (2007). This work has been extended into the nuclear imaging of human brain tracts and networks using diffusion MRI. The Human Connectome Project researchers have recently published a series of articles showing image reconstructions of several human and animal brain tracts (Wedeen, Rosene, Wang, Dai, Mortazavi, Hagmann, Kaas, & Tseng, 2012; Toga, Clark, Thompson, Shattuck, & Van Horn, 2012). Within the Artificial Intelligence (AI) communities, computational models of cognition have now been constructed for the human brain which learn and adapt with experience, closely modelled on known brain neuroscience (Roy, 2012; Eliasmith, Stewart, Choo, Bekolay, DeWolf, Tang, & Rasmussen, 2012).
It is interesting to see how AI researchers define intelligence, for example Poole, Mackworth, & Goebel (1998):
Computational intelligence is the study of the design of intelligent agents. An agent is something that acts in an environment – it does something. The central scientific goal of computational intelligence is to understand the principles that make intelligent behavior possible, in natural or artificial systems. The main hypothesis is that reasoning is computation. The central engineering goal is to specify methods for the design of useful, intelligent artifacts (p. 1).
This recent work and thinking is now moving us into the realm of problem solving and reasoning, and a richer conceptualisation of cognition which extends beyond equating it with intelligence.
Problem Solving
A distinguishing characteristic of life is that it involves the solving of problems (Popper, 1959). Living beings solve problems both phylogenetically, that is, collectively through the adaptation of the species, and ontogenetically, that is direct problem solving through a method of trial-and-error learning. These two types of problem solving give rise, in turn, to structural changes.
The term problem solving thus encompasses a vast range of individual and collective, as well as conscious and subconscious types of behaviour. The literature dealing with problem solving in psychology, however, generally appears as a subsection of the larger body of research on intelligence. Various emphases in the investigation of problem solving can be traced historically. The analysis of problem solving processes was first carried out by Gestalt psychologists, Behaviourists and Associationists (Greeno, 1985).
Before describing current methodology and theoretical approaches to problem solving research, an exposition of the different definitions found in the literature will be presented, such as:
- All cognitive activities are fundamentally problem solving. This view based on the observation that human cognition seems to be purposeful and directed towards achieving goals and removing obstacles to attaining these goals. Some researchers go as far as to view all behaviour as an instance of problem solving. Such an encompassing view of problem solving is, however, regarded by some theorists as too abstract to be of any use empirically (Anderson, 1985; Rips, 1994).
- A problem is a task which involves making a choice where the outcome is uncertain. Problem solving then requires the construction of a mental representation of the problem and the identification of a goal which incorporates certain criteria and also brings alternative concepts related to the criteria into consciousness (Hirschman, 1981).
- A problem is an undesirable situation in which unfamiliar elements cause standard responses to be inadequate. Problem solving thus involves finding appropriate and adequate responses to change the situation (Goldsmith & Matherly, 1986).
- Problem solving is a search through a problem space where the latter consists of various problem states which refer to both physical states and states of knowledge (Anderson, 1985; Greeno, 1976; Kneauper & Rouse, 1985; Newell, 1973; Newell & Simon, 1972).
Problem solving research has thus primarily focused on the following aspects (Chi, Feltovich & Glaser, 1981; Feldhusen, Houtz & Ringenbach, 1972; Greeno, 1978; Hunt & Lansman, 1986; Schultz & Lockhead, 1988; Simon, 1979; Sweller, 1988):
- the phenomena of learning, reinforcement and extinction;
- mechanisms involved in solving well-structured problems, or laboratory tasks, such as the well-known Tower of Hanoi, Missionaries and Cannibals and Waterjug problems;
- the identification of strategic approaches and the circumstances under which they are applied;
- the modelling of expert knowledge storage, or research on the nature of expertise;
- the modelling of knowledge representation including Rumelhart and Ortony’s (1977) representation of the structural aspects of problem solving; Minsky’s (1974) frames as complex data structures for representing stereotypical situations; Schank and Childer’s (1984) scripts, plans and goals; and Siegler’s (I981) rules and principles;
- the exploration of problem solving in semantically rich domains such as: medical diagnosis, accounting and engineering (where specific and general knowledge is required), radiology and physics (where encoding and representation skills seem to be critical), chess (that seems to involve rapid recognition more so than logical analysis);
- the development and testing of production systems; and
- modelling of the understanding of natural language instruction.
This research has resulted in a number of problem solving models, reflecting the following approaches (Feldhusen et al 1972; Greeno, 1978; Knaeuper & Rouse, 1985):
- stages;
- levels of problem solving behaviour;
- the representation of information; and
- lists of problem solving processes.
Some very specific and very general models have been formulated. An example of a specific and detailed model is that of Sternberg (1977c; 1979; 1983). According to him, analogical reasoning can be analysed in terms of the following processes:
(a) encoding, which involves the translation of a stimulus into an internal representation;
(b) inference, which means discovering a rule that relates one concept to another;
(c) mapping, or the discovery of a higher rule that relates one rule to another;
(d) application, whereby a rule is generated that extrapolates from an old concept to a new concept on the basis of an analogy to a previously learned rule;
(e) comparison, which involves the comparison of answer options to an extrapolated new concept to determine which option is closest to the extrapolated concept;
(f) justification, whereby a preferred answer option is compared to the extrapolated concept to determine whether the answer option is close enough to the extrapolated concept to justify its selection as the answer;
(g) the response, which involves the communication of the chosen answer through an overt act.
An example of a general model is that of Hirschman (1981) who identified three steps of problem solving, namely:
(a) preparation;
(b) production; and
(c) judgement.
These very general and specific models are not always ideal for practical application. Although there is no single scientifically meaningful level for the study of problem solving, a number of prominent theorists consequently motivated for the development of theoretical models reflecting an intermediate level of theorising. In other words, models that are general enough to cut across many domains, but are specific enough to be operationalised and evaluated in terms of convergent and discriminant validity.
Although some of the models gave rise to the development of a number of sophisticated computer production systems applying (i) the generate-and-test method; (ii) the heuristic search method; and (iii) the hypothesise-and-match methods (Newell, 1973), research in the area of problem solving is characterised by inadequate conceptualization and a lack of empirical evidence. The further development of appropriate and effective methodology is also a crucial prerequisite for further research.
Problem solving will, for current purposes, be regarded as referring to a broad range of predominantly active intellectual and practical efforts that are directed towards changing a particular state to a different, and possibly desirable or reinforcing, end state. This mainly involves the purposive application of previously acquired knowledge and skills to task materials in a way perceived (meta-cognitively) as being appropriate.
The concept of problem solving is closely related to that of reasoning.
Reasoning
Reasoning is a central everyday activity. The very fact that the concept of reasoning refers to such a wide spectrum of behaviours makes it vulnerable to criticism in terms of its theoretical and practical utility. Some researchers fail to see any advantages in investigating diverse domains under a single heading (Rips, 1984).
In contrast to problem solving research, reasoning research has remained within the realm of inductive and deductive reasoning and has focused on critical questions such as the degree of generativity involved in reasoning, evidence for logical structures underlying thought, explanations for some of the well-researched non-logical biases (such as the atmosphere effect, lexical markings, figural bias and the caution heuristic) and the complexities of natural language.
As with most constructs in intelligence research, the concept of reasoning has been described in various ways and by representatives of different disciplines, which even include economists and mathematicians. These two disciplines both stress the role of decision making through reasoning and could well have relevance for psychology (Evans, 1982).
Some theorists conceive of reasoning in very general terms, for example, encompassing almost any process of forming or adjusting beliefs and nearly synonymous with cognition itself (Rips, 1984). An example is the view that reasoning refers to the process by which people evaluate and generate logical arguments. Reasoning is also described as goal directed mental activity aimed at arriving at a valid conclusion on the basis of a given set of facts, arguments, premises or reasons (Erickson, 1974).
An additional dimension often referred to or implied, is that of generativity or creativity (Holyoak & Nisbett, 1988). According to this view, reasoning involves the use of rules in drawing inferences that extend one’s knowledge. This view emphasizes the unique nature of reasoning in terms of its centrality in explaining human behaviour and its productive and creative capacity.
The above views and the subsequent research on reasoning are regarded by some as limited in that it has to date primarily focused on deductive reasoning (e.g. syllogisms), and inductive reasoning (studied by means of geometric shapes, dot patterns and schematic faces). Broader, more naturalistic theorising is needed.
A more detailed delineation of the concept of reasoning is provided by Sternberg (1986) who regards reasoning as the controlled and mediated application of selective encoding and selective comparison, which are essentially inductive in nature, as well as selective combination, which is essentially a deductive process. According to Sternberg, tasks that involve none of these processes do not involve reasoning per se. Tasks that involve some of these processes involve reasoning to the extent that the application of the processes is not automatic.
The content dependent nature of reasoning has sparked much interest among researchers (Evans, 1982; Rips, 1994). When defining the concept reasoning, some reference should thus be made to task content.
From the definitions to be found in the literature, reasoning can thus be described in terms of the degree to which mental activity involves the controlled selection of content (facts and premises) structured in memory, and the recombination of its elements according to certain rules in order to generate probable and necessary conclusions, or inferences, referred to in the literature as inductive and deductive reasoning respectively.
Deductive and Inductive Reasoning
Reasoning is commonly divided into the inductive and deductive types (Evans, 1982). Deductive reasoning is described in terms of proofs in which a conclusion is derived/deduced/follows from a set of given premises. Inductive reasoning attempts to derive empirical generalizations and probable premises from exemplars of phenomena (Haig, 2005). Concerning the validity of an argument, inductive inferences are strictly, not valid whereas deductive inferences are. The validity of an argument stays unaffected by whether the premises and conclusions are true or false. Invalid arguments may well lead to true conclusions. Logical systems comprise sets of rules for making valid deductions. The distinction between inductive and deductive reasoning merely reflects a philosophical difference in the criteria for the evaluation of an argument (where an argument takes the form of a set of sentences or premises, followed by a conclusion). The use of these criteria does not demonstrate a psychological distinction between the two types of reasoning because there are no grounds for assuming that different psychological processes are involved. However, if there is only one process, the details of this process should be delineated. Tasks that involve both inductive and deductive processes include many daily tasks, scientific inference and mathematical and logical proofs.
Having briefly discussed the concepts of problem solving and reasoning, the relationship between the two constructs will now be explored.
The Relationship Between Problem Solving, Reasoning and Logic
Because solving problems usually involves reasoning processes or vice versa (depending on the description of the concepts), reasoning as well as logic will be considered here.
The concepts of reasoning and problem solving have both been criticized for being too general and abstract. The range of behaviours they cover is so wide that it limits their practical usefulness. Some problem solving studies do, however, focus on clear-cut tasks. Most reasoning models are indebted to problem solving theory. Some researchers, however, prefer the concept reasoning to that of problem solving as being empirically more useful (Rips, 1994).
The concept of “logic” refers to the construction of formal systems by which given formulae can be transformed according to rules governing the manipulation of symbols. It can be seen as a sub-discipline of philosophy and mathematics and formally specifies criteria for an argument to be logically correct. A logical system thus comprises rules of inference which permit true conclusions to be derived from true premises and in this sense provides a normative model for reasoning behaviour (Anderson, 1985).
It is, however, a misconception to regard mental operations as fundamentally logical in nature. In fact, much of human thinking lies outside the boundaries of established formal logic. In contrast to the subtleties and complexity involved in human thinking, only two values, namely true and false, are permitted in standard logic (Wason & Johnson-Laird, 1972).
A proposition can be seen as a statement with truth value. Premises have truth values and are therefore propositions. The elements of premises, for example P and Q, are component propositions. The relationship between propositions is such that they can be combined to form new ones. Formal validity in logic is reflected by a form in which instances have true conclusions if the premises are true. Where the conclusions are not formal consequences of the premises, inferences may still be regarded as valid in the derivative sense if the conjunction of premises with certain conceptual truths formally imply certain conditions.
The question of whether deductive logic is a natural characteristic of human thought depends on the paradigm adopted. A rationalist approach, such as that presented by Kelly in 1955 (Evans, 1982), describes people as scientists who continually construct theories, make predictions and collect evidence. This approach assumes that people possess some system of deductive logic.
Rationalism is manifested in a number of approaches in reasoning research. Behaviourists, however, interpret behaviour as based on a personal history of reinforcement rather than on an internalised logical system. Many intermediate positions can also be identified. There are various systems of formal logic and the criteria of formal logic are relatively simple compared to the complexities of natural language (Evans, 1982).
Formal logic can thus be criticised for not accommodating language factors. Certain features of formal logic are also linguistically meaningless because logic refers to structural aspects regardless of the semantic correctness of a conclusion (Evans, 1982).
Findings regarding the dependency of thinking processes on content, further clarifies the relationship between logic and reasoning. This content dependency of reasoning processes is reflected by findings indicating for example that (a) thematic or known content seems to affect logical performance; (b) beliefs associated with certain contents may bias responses; and (c) semantic contexts influence interpretation and inference. Researchers have found that content which pertains to subjects’ personal experience may facilitate performance by triggering the use of existing representations and knowledge systems. Reasoning responses to known content are simply appropriate to the subject’s experience and may or may not be according to principles of logic. Known content does therefore not necessarily indicate reasoning ability.
This raises the question of whether all reasoning is simply a function of specific experience or whether it can be interpreted in terms of an underlying system of reasoning competence which is content independent but influenced and modified by specific content. No conclusive evidence is available on this matter as evidence on logical reasoning is insufficient to prove the existence of underlying logical competence (Evans, 1982).
The generalisation hypothesis seems to be an appropriate explanation of many of the research findings: There is the possibility that subjects simply generalise from previous experience. Various research studies have verified this by indicating that the content and direction of reasoning processes are highly stimulus bound. Evidence for formal logical competence is surprisingly lacking. This finding contradicts Piaget’s view of adult intelligence as logical. It does, however, fully support Allport’s view that the nature of cognition is content specific.
One interesting and somewhat puzzling finding is that when subjects are asked to give a verbal explanation of their reasoning responses, their verbal protocols seem to be constructed as a justification of their behaviour, and reveal distinctly logical thought (Evans, 1982). This discrepancy between performance and introspection raises the question of why this capacity for logical reasoning is not used when first solving a problem. Could it be that the greater self-awareness and metacognitive involvement of such explanations and justifications provide easier access to the rules of logic?
Existing research findings therefore seem to suggest that logical competence is not necessarily reflected in reasoning performance and that it is affected by a number of variables which influence the application of this competence. Task interpretation and cognitive style are given as examples of such moderator variables. Content also plays a central role in reasoning, regardless of the existence of underlying logical competence.
The relationship between formal logic and reasoning can be summarized with the statement that logic provides the principles by which the validity of deductive reasoning can be evaluated.
A Theory-Based Model of Cognitive Processes
A powerful research catalyst in the field of cognitive psychology can thus be found in questions regarding the structure of the mind and its bearing on reasoning and problem solving. Research questions on the topic gave rise to the development of a number of theoretical and methodological approaches – all of which are characterised by specific theoretical shortcomings. The proposed model represents a systems approach concerned with function as a basis for understanding structure, thereby accommodating both the process and structural approaches. This model is thus conceived at the interface between experimental and differential psychology and contributes towards the body of research emphasising the importance of increasing integration that is emerging as a trend in cognitive psychology.
Within the model, five cognitive processes are envisaged as operating on four “levels” or “modes” of processing, The processes reflect functional unities and are regarded as having descriptive rather than explanatory value. They are defined and compared with similar constructs in the literature in an effort to expand the existing nomological network. The processing constructs are represented as overlapping fields of a matrix, a view comparable to that of “holons”, which is a term coined by Wilber (2000) to indicate the hierarchical organisation of the universe where evolution involves the emergence of increasingly transcendent yet inclusive systems. A degree of overlap between consecutive levels of systems or functional complexity is thus involved, where higher levels of organisation are dependent on preceding levels. This explanation offers a more elegant theoretical perspective than that of the stages, phases and categories models in intelligence research. Figure 1 shows the holonic representation of processes.
In Figure 1, Metacognition is both the encompassing awareness and process that is a prerequisite for effectiveness / capability (but one can still explore, analyse, structure, transform without a great deal of metacognitive involvement). Metacognition is involved with all the processing categories, guiding each via specific criteria which form the basis for the Cognitive Process Profile (CPP) assessment. These criteria are shown in Figure 2.
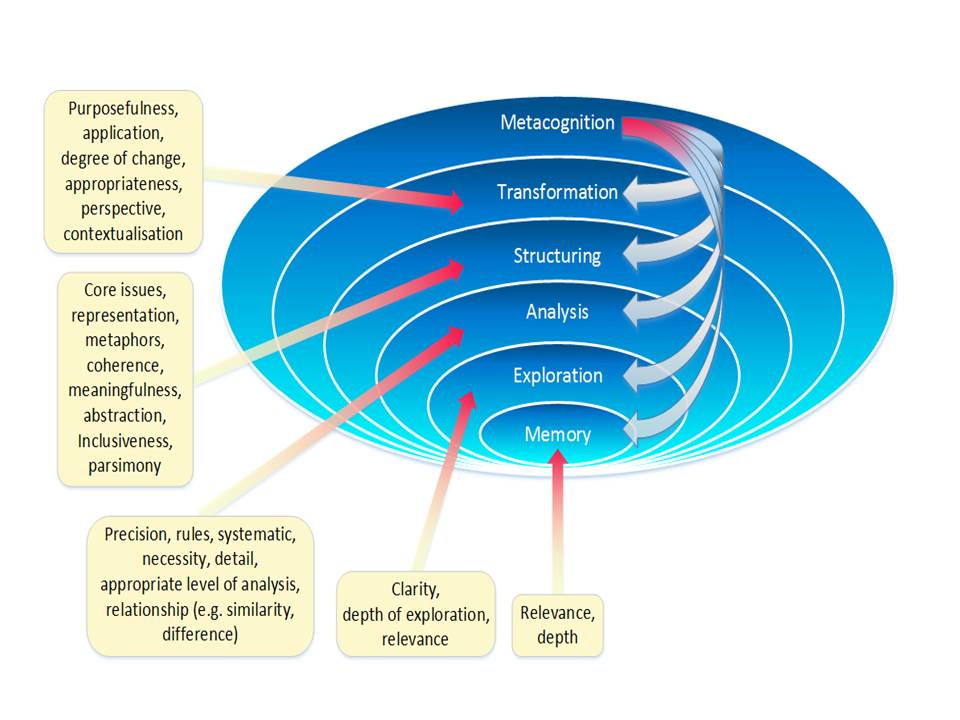
Figure 2: The Metacognitive Criteria Guiding Each Process
The four levels or ‘modes’ of processing are defined as:
Process |
Description |
Performance |
Dealing with task material (external focus) |
Metacognitive |
Guiding performance processes in a self-aware manner (internal focus) |
General/Rule |
Knowledge and experience of a content domain organised as rules |
Subconscious |
Vague awareness and intuitive insights derived implicitly from knowledge, experience and emotions |
Table 1: The four levels/modes of processing
Figures 3, 4, and 5 show how within the processing model, cognition depends upon context.
The effectiveness of the contribution of processing activities at each of these levels / modes, depends on the cognitive requirements posed by the specific context. For purposes of illustration, 3 such environments and their processing requirements are graphically represented below, namely:
- complex environments
- structured environments
- innovative environments.
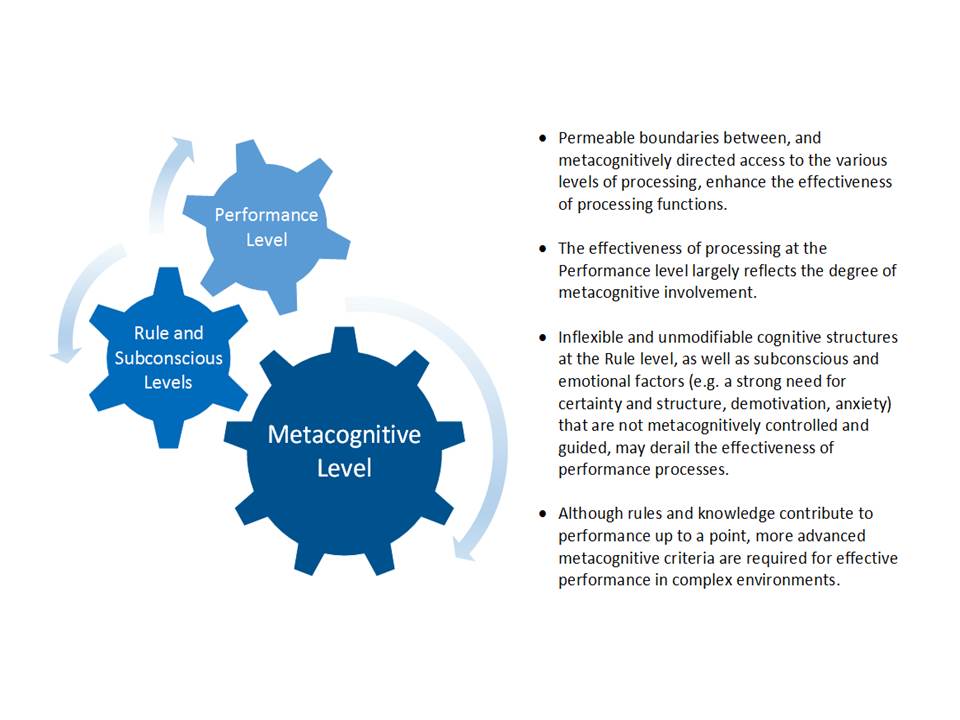
Figure 3: Complex environments (unstructured, vague, ambiguous, unfamiliar environments, cognitively challenging problem solving and contextualisation).
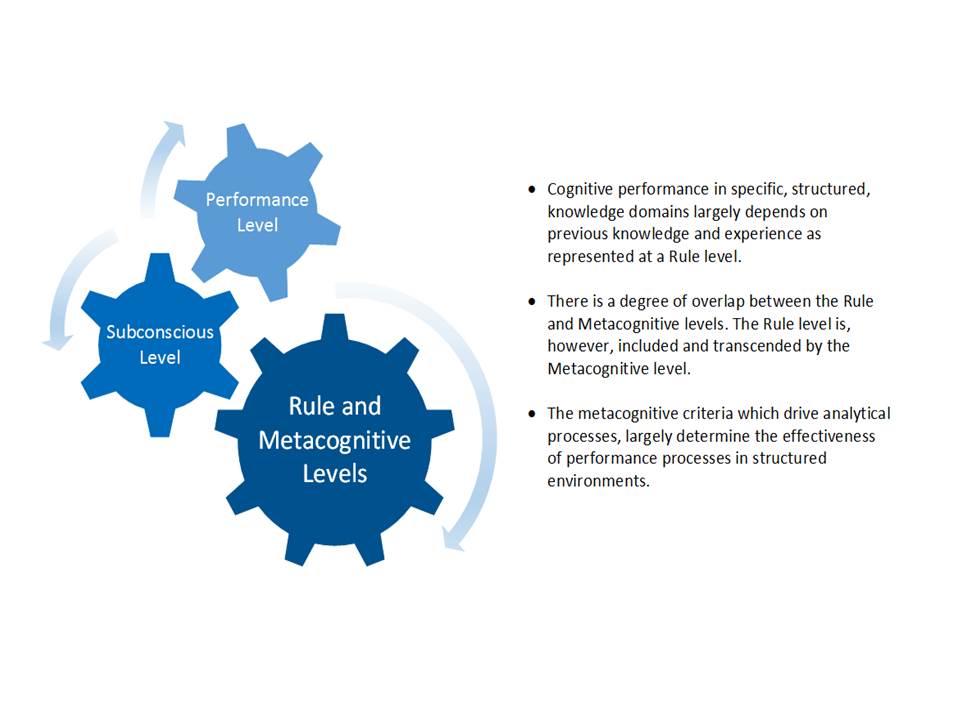
Figure 4: Structured contexts (based on knowledge and experience).
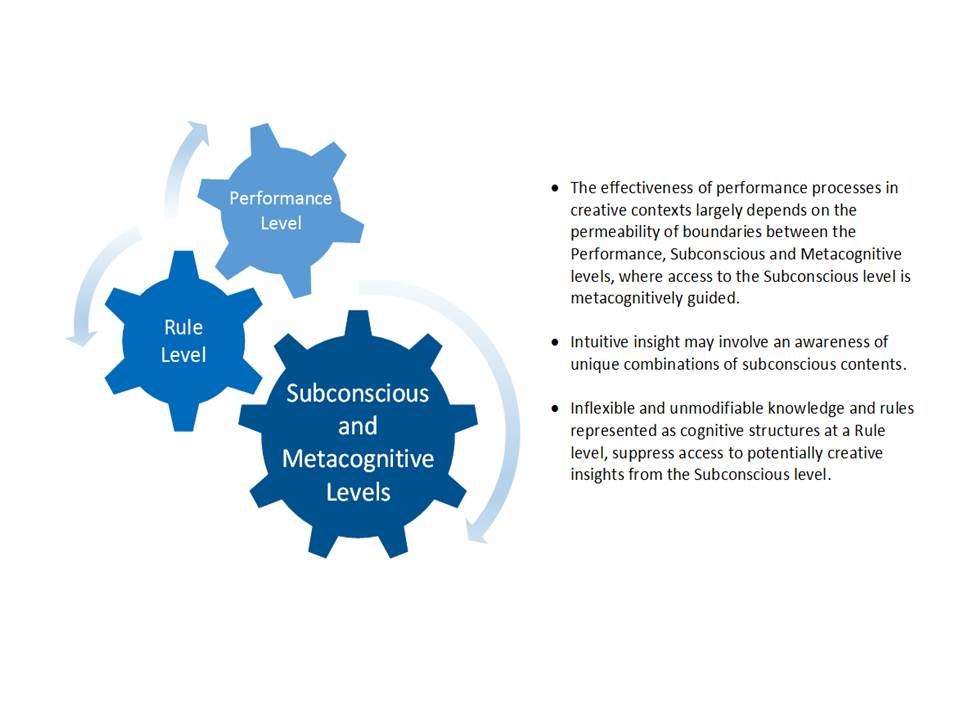
Figure 5: Innovative contexts
The processes, as functional categories, and the notion of levels can be described as follows:
Focusing and selecting involve the application of processing activities to explore complicated perceptual and/or conceptual configurations according to criteria of relevance in order to select particular elements for further processing. This can be viewed as a rapid search through a mass of relevant and irrelevant data to select that which is relevant. Focusing and selecting is mostly guided by the metacognitive criteria of relevance, familiarity, the degree or depth of exploration and clarity.
Existing theoretical constructs of processing activities that can be related to the process of focusing and selecting are: encoding (Carroll, 1981; Sternberg, 1977a); selective encoding (Sternberg, 1986); problem space exploration (Feuerstein, Rand, Hoffman & Miller, 1980; Spearman, 1923; Sternberg, 1977c; 1979; 1983; 1986; Sternberg and Rifkin, 1979; Sternberg & Smith, 1986; Wagner & Sternberg, 1984; Whimbey & Lockhead, 1980; Whiteley & Barnes, 1979); attribute discovery (Sternberg, 1977c); problem detection and recognition (Baron, 1982); detection (Resnick & Glaser, 1976); feature scanning (Resnick & Glaser, 1976); and apprehension (Carroll, 1981; Spearman, 1923).
The processing construct of linking can be described as the application of processing activities to perceptual and conceptual configurations in order to identify the elements involved, the common properties and/or relationships between these elements through the comparison of their structural characteristics. The relating, or linking, of information structures often precedes, and hence forms a basis for, most other processes. Linking and other analytical processes are guided by metacognitive criteria such as: observation of rules, detail and precision, accuracy, being systematic, the most appropriate level of specificity or generality and interrelationship (e.g. similarities, differences, degree of matching).
Existing theoretical concepts that can be compared to that of linking are: inference (Spearman, 1923; Sternberg, 1977b; 1977c; 1983; 1986; Sternberg & Smith, 1986; Wagner & Sternberg, 1984); processes such as comparing, associating, combining, transferring and matching (Cronen & Mikevc, 1972; Sternberg, 1977c; 1979; 1983; 1985; Whiteley & Barnes, 1979); selective comparison and selective combination (Sternberg, 1983; 1985; 1986); enumeration of possibilities (Baron, 1982); production (Hirschman, 1981); education of relations (Spearman, 1923); Simon and Kotovsky’s (1963) three processes involved in the solution of series problems, namely the detection of relations, the discovery of periodicity, and the completion of pattern descriptions; solution through analogy (Schultz and Lockhead, 1988); Burt’s (1949) apprehension and combination of relationships; similarity judgements (Estes, 1986); and the understanding of relations (Spearman, 1923).
Inductive reasoning tests such as analogies, series completions and classifications, and deductive reasoning tests such as linear, categorical and conditional syllogisms primarily require relational thinking. Thurstone (1938 in Colberg, Nester & Trattner, 1958) described induction as the finding of a rule or a principle and suggested that it might be comparable to Spearman’s g, a concept which is closely associated with intelligence testing.
The processing construct of structuring involves the application of processing activities to perceptual and/or conceptual configurations so as to order or group their elements to represent particular relationships and form coherent structures according to criteria of meaningfulness. Structuring activities are involved with problem representation, the subsequent utilisation of such representations, the modification of inadequate representations and the integration of knowledge structures. It may range from conceptualising simple models and ideas, to complicated and integrated networks and may incorporate aspects of other processes. The relevant metacognitive criteria that guide structuring and integration processes include: coherence, meaningfulness, abstraction, representation, pattern, core elements, inclusiveness and simplicity.
A variety of processing activities referred to in the literature can be associated with structuring. These include: representing a structure (Hirschman, 1981; Whimbey & Lockhead, 1980); co-representation formation (Carroll, 1981); defining a problem (Feuerstein et al., 1980; Resnick & Glaser, 1976); mapping (Sternberg, 1988; Sternberg & Smith, 1986); Baron’s (1982) stages of the enumeration of possibilities and reasoning; understanding (Greeno, 1978; Larkin, 1985); as well as processing activities that are often mentioned in cognitive psychology, such as categorising, grouping, ordering, chunking, organising, conceptualising and formulating.
The concept of “structuring” is also central to the schema theories that have contributed greatly to the development of Artificial Intelligence and simulation or production programs. It is also implied by most of the cognitive style theories formulated in terms of concepts such as cognitive complexity, conceptual differentiation, category width and equivalence range, conceptual integration, analytic versus relational categorisation, compartmentalisation, preferred level of abstraction and levelling versus sharpening, as was mentioned by Wardell and Royce (1978). Expert-novice studies, and in particular those in the semantically rich domains of Physics and Radiology, have indicated that expertise is primarily characterised by superior problem representation, which can be seen as a structuring activity.
The processing construct of transformation involves processing activities applied to mental representations for the purpose of changing structural elements to establish and/or change inter-structural links via logical and lateral reasoning processes. Quantitative and qualitative transformations can be identified. Quantitative transformations involve a meta-cognitively directed series of linking steps applied for transformational purposes, mostly of a rigorous logical or convergent nature. In a qualitative transformation the newly created structure cannot be linked sequentially to the previous one. Qualitative transformations often involve intuitive or abstract conceptual processing. Both metacognitive awareness and the subconscious may play a role. An example is the particle versus wave theories of light in Physics. The transformation of elements or relationships in problem solving often requires divergent thinking. Transformation usually involves complex processing and is therefore more integrated in terms of the variety of cognitive processes and levels involved. It strongly capitalises on metacognitive guidance of the thinking process via criteria of: purposefulness, difference/novelty/creativity and applicability.
The process of transformation can be related to the following concepts found in the literature: Carroll (1981) referred to the transformation of a mental representation; Baron’s (1982) stage of the enumeration of possibilities includes the transformation of structures; Guilford (1967) mentioned the product of transformation; and Greeno (1978) described transformation as the construction or generation of new relationships and components.
The processing construct of retention and recall involves the application of processing activities to perceptual and/or conceptual configurations for the purposes of both storing and retrieving information. Storing and retrieving, or memory processes may, however, involve different mental mechanisms. It is guided by the metacognitive criteria of relevance, significance, importance, familiarity and relationship.
Numerous research findings point to the centrality of memory functions in cognitive competence (Burt, 1949; Carroll & Maxwell, 1979; Estes, 1986; Eysenck, 1986; Larson & Saccusso, 1989).
According to Snow (1979), no other area in cognitive psychology has received the amount of research attention that memory functions have. This may be because working memory plays such a central role in thinking, all other processing activities probably include memory functions to some extent (Carroll & Maxwell, 1979). Although the processes of retention and recall are discussed jointly, they are, in all probability, fundamentally separate processes. Findings indicating recall as a reconstructive process support this view. Horn (1986) also observed that the retrieval of information seems to be independent of the storage thereof and has stated that individual differences on one of these functions is not fully predictive of differences on the other. Retention and recall are, however, postulated as a single processing construct on the basis of their functional nature and the fact that retention is a precondition for recall.
A literature review of memory research reveals certain trends. Early research attempts focused on quantitative phenomena in memory performance. A typical example of this type of research is the learning and forgetting curves researched during the 1950s and 1960s. Contemporary memory research emphasises the mechanisms involved in memory performance. A number of theorists are of the opinion that memory is an associative process (Anderson, 1975) and certain production systems regard productions as equivalent to associations. In addition to association based on content, covariation is also regarded as a basic relational mechanism.
Theorising on memory focuses predominantly on the construction of memory models. Memory models are dominated by the concept of stores and the transfer of information between them. Stage, level and trace theories have also been developed. It is widely accepted that memory functions can be described in terms of three levels of storage, namely sensory stores, short term memory and long term memory (Craik & Lockhart, 1972). This notion of stores is incorporated in the multi-store models. Various store models reflect the spread of activation idea (Anderson, 1985; Gitomer & Pellegrino, 1985). Spread of activation takes place along a network of paths in which concepts are represented as nodes linked to represent certain types of relationships.
Stage theories describe perception in terms of the rapid analysis of stimuli in consecutive stages. Sensory features are focused on first and then compared with stored abstractions. However, several findings indicate that perception occurs at meaningful, deeper levels first (Craik & Lockhart, 1972). Stage theories are associated with the multi-level view. The levels view focuses on types of analysis rather than on kinds of memory. According to this view the more superficial properties of a stimulus are analysed first and the more profound properties later.
The “deeper” the analysis of an item is, the longer its trace (Treisman, 1979). Original views of levels have been extensively modified and contemporary approaches include the idea of parallelism as opposed to the serial-processing theory on which the levels notion was initially based (Kohlers, 1979). The trace durations of information on various levels, which are held by different memory stores, have been extensively researched. A memory trace is seen as a record of experience and is represented internally as an active configuration of primitive properties such as the item’s sensory features, modality and relations.
Elaborated traces provide alternative sources of activation, thereby contributing to the spread of activation. Three theoretical stances describe the degree of generativity involved in recall. These are the reappearance hypothesis, the constructive theories and the reconstructive theories. According to the reappearance hypothesis, remembering involves locating a memory trace of a sensory experience and bringing its contents to consciousness. The constructive theories view recall as the reviving of stored material including sensory experience, existing knowledge structures and environmental features. According to reconstructive theory, remembering involves the reconstruction of past events on the basis of current schemata in memory (Estes, 1986; Spiro, 1977).
The way in which information is stored in the brain also remains unclear, though various metaphors have been used to describe it. Computer storage and holographic storage are examples.
According to Hintzman (1990), researchers hope that models developed by means of connectionism will eventually serve as a bridge between neurobiological evidence and behavioural phenomena. Although this goal seems ambitious at present, explicit comparisons are already being made between the circuitry of the hippocampus and certain connectionist networks. However, the complexity of the neural system remains a stumbling block.
The processing construct of response refers to the application of processing activities for the purpose of behavioural expression. Although response can possibly be regarded as an additional processing construct that can be incorporated into a model such as the proposed one, it has not been included or measured. This is because the measurement of the other processes necessarily requires a behavioural response. Such a construct would therefore be extremely difficult to isolate experimentally. The exploration of the nature of this process should, however, constitute a future research goal.
It is hypothesised that the proposed processes operate on different “levels”, or in different ÏmodesÓ, namely the performance, metacognitive, general or rule, and subconscious levels / modes. The differentiation of levels of processing is common in cognitive theory and has been referred to by prominent theorists such as Vygotsky (Van der Veer & Van Ijzendoorn, 1985), Strayer and Kramer (1990), Schneider (1985), Treisman (1979) and Keating (1984).
The proposed four levels, or modes, of processing can be described as follows: Processing activity on a performance level focuses on the task material rather than on the mental activities. This level of processing has been referred to by Evans (1982) and Sternberg (1981; 1983; 1985; 1988). The metacognitive level implies self-awareness and tends to be of a relatively complex nature. Processing usually occurs on this level when the problem solver directs, monitors, evaluates and plans the processing that is taking place on the performance level. Processing on the metacognitive level is usually of a more integrated nature in terms of the variety of processing activities used. Metacognition can thus be regarded as a skill by which the problem solver directs his or her own thinking processes (Chipman, Segal & Glaser, 1985; Embretson, Schneider & Roth, 1986; Kneauper & Rouse, 1985; Meichenbaum, 1980; Sternberg, 1977b; 1988; Sternberg & Spear, 1985). Sternberg specifically described meta-components as elements of adaptive intelligence, regardless of the cultural context, thereby emphasising the concept’s universal value (Sternberg, 1983; 1984; 1985; Sternberg & Spear, 1985).
A large number of dichotomous classifications distinguish between well-ordered processes on the one hand and a confusion of cognitive activity on the other (Neisser, 1967; Estes, 1986). These two dimensions are reflected by the general or rule, and the subconscious levels of the proposed problem solving model. On a general or rule level, specific procedures are organised and structured as rules whereas on a subconscious level activity is of a rather unstructured nature. Researchers who have implicitly or explicitly referred to processing on a general or rule level include Cronen and Mikevc (1972); Evans (1982); Geman (1981); Henle, 1962; Rumelhart and Ortony (1977); and Sternberg (1986). Those who have implied or mentioned processing on a subconscious level include Anderson (1985); Ellis, Meador and Bodfish (1985); Evans (1982); Gitomer and Pellegrino (1985); Guilford (1967); Sternberg (1981; 1983; 1986); and Vygotsky (1978).
Thus, the “levels of processing” aspect of the model incorporates two main dimensions:
- one that reflects the degree of structure and order; and
- one that reflects the internal or external focus of the mental activity.
The degree of awareness that can be regarded as a third dimension, is largely implied by the metacognitive and general or rule, poles of the two dimensions. Awareness is, however, an individualised phenomenon reflecting the “degree of intelligence” (as a descriptive concept) involved in processing activities. On a subconscious level awareness can, for example, be reflected by the degree of openness to intuitive insights and on a general or rule, level, by an analytical approach.
As already indicated, the criteria applied for the identification of the proposed constructs are (a) its purpose in terms of application; (b) its generality; (c) its necessity; and (d) whether it is consciously or automatically applied. These four criteria limit the arbitrariness involved in the categorisation of basic processing units which are of a fluid nature. And, as already explained, the proposed processing constructs can be represented as overlapping fields of a matrix incorporating processing activities identified in the literature and major areas of task contents, graded according to the complexity involved. The following abbreviations are used in Table 2 to indicate the processing constructs: F for focusing and selecting; L for linking; T for transformation; S for structuring; M for retention and recall; and R for response.
Existing Processing Constructs Identified in the Research Literature |
Levels of Increasing Complexity |
|||||
Elements |
Relationships/rules |
Patterns |
Meaningful wholes/systems |
Dynamic systems |
Chaos and emerging pattern |
|
Attention allocation, focus |
F M |
F M |
F L M |
L S M |
S T M |
T M |
Search, scan, explore |
F M |
F L S M |
F L S M |
L S T M |
S T M |
T M |
Recognise, discriminate, choose, identify, select, eliminate, ignore, encode, etc |
F L M |
F L S M |
L S T M |
L S T M |
S T M |
T M |
Compare |
F L M |
F L S M |
L S M |
L S T M |
S T M |
T M |
Relate, combine, add, associate, link |
L M |
L S M |
L S M |
S T M |
S T M |
T M |
Infer, deduce |
L S M |
L S M |
S M |
S T M |
T M |
T M |
Organise, arrange, order, group, chunk, classify, categorise, systematise, structure, represent |
L S M |
S M |
S M |
S M |
STM |
T M |
Integrate, synthesise, unite, assimilate |
S M |
S M |
S M |
S T M |
T M |
T M |
Formulate, define, conceptualise, concept formation |
S M |
S M |
S T M |
S T M |
T M |
T M |
Change, manipulate, accommodate, transfer, tune, transform, create |
S T M |
S T M |
T M |
T M |
T M |
T M |
Rehearse, practice, link |
S M |
S M |
S M |
S T M |
T M |
T M |
Store |
S M |
S M |
S M |
S T M |
T M |
M |
Recall, retrieve |
M |
M |
M R |
M R |
T M |
M |
Apply, implement, communicate |
M R |
M R |
M R |
M R |
T M R |
T M R |
Table 2: Processing activities represented as overlapping fields of a matrix
Note: F for focusing and selecting; L for linking; T for transformation; S for structuring; M for retention and recall and R for response.
The Cognitive Process Profile (CPP)
The CPP is the practical application designed to assess the specific processes and concepts shown in Figure 1. It measures intellectual functioning in terms of constructs such as judgement and decision making, strategising, generalist versus specialist orientation, creativity, complexity preferences and other thinking and problem solving factors related to professional, managerial and executive functioning. It is an advanced computerised assessment technique; using simulation exercises. Subjects are monitored in terms of their preferences and capabilities in exploring, analysing, structuring, transforming, remembering, and learning information and making decisions, or exercising their judgement. The results can serve both as a source of personal understanding and development, as well as being linked to job-related performance. Figure 6 summarises the key processing components and styles.
The CPP assessment consists of a task requiring the deciphering of hieroglyphic messages. It was designed to externalise and track each of the thinking processes specified in Figure 1, and their many subcomponents. While completing the test, a person explores, links, structures, transforms, remembers, clarifies and monitors his/her actions on the computer screen using a computer mouse. All the ÏmovementsÓ made on the computer screen are saved as the person traverses the test. At the end of each task, the person provides his/her interpretation of the symbolic message (normally a one-line statement) by keying it into the computer. A scoring and statement parsing system subsequently integrates all these movements and ‘story’ interpretations, which are subsequently analysed using more algorithms to produce the CPP report. For the CPP to measure the various concepts detailed in the different sections of the report, the cognitive processes are grouped and analysed in many different ways. These intricate groupings often overlap, and the analysis performed by the software is highly complex.
For more detail on the attributes and processes assessed by the CPP, there are several example reports available for download from http://www.cognadev.com/products.aspx?pid=1.
Validating an Integrated Theory of Cognition and the CPP
At this juncture, it is useful to consider how to answer the question: “So you have a theory of cognition and an assessment tool, the CPP, which you claim provides an assessment of an individual’s cognitive styles and processes; how might we be assured that the ‘knowledge claims’ comprising the theory and CPP scores/style-assignments are justified?”. In essence, how to determine the validity of either the theory or the CPP? Here it is important to understand the process of theory-generation, and the difference between description and explanation. Haig (2005, 2013) has set matters out in great detail; here we just wish to outline the essentials in a more brief and ordered exposition.
Step 1: Phenomenon detection
Bogen and Woodward (1988; Woodward, 1989, 2000) initially set out the core arguments concerning phenomena. That is, the initial generating stimuli for theories are not data, but phenomenal observations. As Haig (2005) puts it:
Phenomena are relatively stable, recurrent, general features of the world that, as researchers, we seek to explain. The more striking of them are often called effects (p. 374).
We first have to detect a phenomenon, usually by simple observation of some consistent occurrence or set of events. Detection may be subjective, but for something to be classed as a ‘phenomenon’, it must also be readily observable by others.
Step 2: Description
During the detection phase, observers seek to describe the phenomenon and its qualities more comprehensively. The environmental and situational features surrounding its observation and detection may be formalised.
Step 3: Causal Explanation
Observers now seek to explain the causes of the phenomenon. What’s important here is that observers move beyond phenomena detection and description. It is now established that a phenomenon exists, and can be described in some detail. No more efforts go into detection; rather, the focus now is on answering three questions: “how, when, and why does it occur”? This is where observers begin to formulate theories which attempt to explain the phenomenal occurrence, generating rules for instantiation of the phenomenon, models, and predictions, which can be formally evaluated by experimentation and/or further observations in order to establish whether the understanding of cause is correct or mistaken.
Those three simple steps define the scientific method; no more, no less. It is important to note that in the above description of the scientific method, no distinction is made between quantitative and non-quantitative science. The introduction of a quantity of anything is a very specific feature of a theory, which brings with it certain requirements for observations and theory-test.
Where things become ‘interesting’ are in step 3; the exploration of causality. Theory generation, specification, prediction, test, and evaluation is nearly always an abductive process. It involves proposing initial theories, making predictions, testing those predictions, making relevant observations, and evaluating the consequences in terms of error. The theory is then adjusted or a new theory is created if needs be, incorporating the new observations, collecting new data, evaluation, and so on. It is an iterative process; embodying Peirce’s (1931-1958) concept of abductive reasoning (i.e. the notion of inference to the best explanation). It is how physics and the natural sciences have progressed over 2,000 or more years of observation and investigation (Stehle, 1994).
So, where might we locate the first author’s model of cognition with respect to the steps above? Clearly, we all recognize the meaning of that word cognition, and how and when we can observe it in ourselves and others. Our descriptions may be varied, broad, and diffuse, but the core meaning “involving thought” is the universal here. In short, the phenomenon has been detected and variously described. The model for cognition set out here is simply a more detailed description of the phenomenon and potential ancillary constituent components. But, it might be argued that the model set forth here goes beyond mere description; it is seeking to explain the phenomenon we observe as “cognition”. The difficulty is that Figure 1 is a schematic; it proposes processes and functional relations between them in a generic, descriptive fashion. The model is fundamentally descriptive, but nevertheless does embody the notion of causality within the systems-interaction framework.
Is it valid? To the extent that the model is a plausible description of an observed phenomenon, yes. But, the question: “Is it the best explanation?”, poses the challenge of construing how to determine which of a set of competing models of the phenomenon ‘cognition’ provides the most accurate explanation, which can be addressed in terms of a number of meta-theoretical criteria.
Meta-theoretical criteria in model construction
The formulation of the proposed theoretical model took place according to a number of meta-theoretical criteria, including: parsimony and the level of theorising; a theoretical foundation for the operationalization of constructs; the falsifiability of the model; the structural adequacy of the model; heuristic usefulness; specificity; empirical adequacy; and practical utility.
The theoretical requirement of parsimony or simplicity, is an important criterion to be considered in theory construction. However, there generally is a trade-off between the parsimony and completeness of a theoretical model. Detterman (1984) emphasised parsimony as the most important criterion in theory construction, particularly in areas of relatively weak theorising. According to him, parsimonious models should first be invalidated before more complex explanations are proposed.
The basic unit of analysis that is adopted for theoretical purposes reflects the particular level of theorising, or the degree of molarity or molecular structure of the concepts involved. Theorising in cognitive psychology takes place on several levels, ranging from an elementary to a higher, more integrated level.
Not all existing models satisfy this criterion of parsimony. Although there is no “correct” level, Sternberg (1988) and Detterman (1984) respectively motivated for theorising on an intermediate and a more general level. The theoretical ideal of parsimony, as well as research findings supporting the utility of a small number of generalised constructs, leads to this same conclusion.
A number of theoretical models reflect either a very general level of focus, or a focus on a micro-level in processing. The three-stage theories of problem solving are examples of theorising on a very general level. Much of the information processing research using reaction time methodology, as well as some of the domain specific processing models, can be criticised for being too specific. Sternberg’s (1977b) Triarchic Theory of intelligence is an example of such a specific model. It has subsequently been criticised for being complicated and over-inclusive (Eysenck, 1984; Jackson, 1984) and for containing internal conflicts (Baron, 1984; Economos, 1984).
The model proposed in this study attempts to meet the criterion of parsimony by incorporating only a few basic processes on an intermediate level of theorising. The constructs or cognitive processes, cut across various problem solving domains. This is comparable to the level at which Sternberg (1977a) conceptualised his cognitive strategies, i.e. the level he regarded as the most viable level for research. It is not claimed here that the proposed constructs are elementary processes, but rather that they reflect a level of analysis that is useful for understanding individual differences in processing activities.
The theoretical foundation of a model on which the operationalization of constructs is based, is of critical importance. Theory informs the way in which constructs are linked to observable data, i.e. test items or tasks. A number of theoretical models have been criticised for not providing motivation for the conceptualisation of theoretical constructs, by instead allowing methods to dictate theories (Sternberg, 1984). The availability of the factor analytic technique has, for example, tempted many researchers to allow it to guide their research.
The formulation of the proposed model was, however, not dictated by the availability of methodology. It involved the theoretical integration of existing research findings from various schools of thought; an intuitive process; the application of task analytic procedures; and a combination of the multivariable factor analytic design with the classical single variable design within the framework of the analysis of variance technique.
The operationalisation of the proposed theoretical constructs plays an important role in the process of model building. In order to formulate test tasks or items by which constructs can be evaluated, a careful clarification of the theoretical criteria involved, is required. Many existing attempts to operationalise constructs and develop items lack this type of theoretical clarification. The analogical items so popular in intelligence research still resemble those used in the early twentieth century, even though these items have been frequently criticised for being a-theoretical (Sternberg, 1988; Whiteley, 1973). Contemporary constructs and testing techniques still lack the required theoretical description and research into the processing activities involved.
For purposes of the proposed model, certain criteria were devised and applied in the operationalization of constructs to differentiate and categorise the rather integrated and somewhat overlapping thinking processes. These were:
- the purpose for which processing activities are applied, or their functional value;
- generality (Sternberg, 1986), or whether the processing activities are usually associated with a particular process;
- necessity (Sternberg, 1986), or whether the activity is essential for the performance of a particular process; and
- whether a process is consciously, as opposed to automatically, applied.
In terms of the falsifiability of the theoretical constructs or theory, it should be noted that although construct definitions and theories cannot be falsified, models containing these constructs can generate falsifiable predictions in terms of construct validity.
The present study applied this falsification approach to the proposed model, which is, very importantly, conceptualised as a self-contained structure. The model was tested according to a multitrait-multimethod technique incorporating a task analytical approach and a model fitting technique to determine construct validity.
Given that very little is known about thinking processes and brain activity, definitions of cognitive processes should be as specific as possible in terms of their functions, their interrelationships with other theoretical constructs, the nature of the information involved, the representation of the information, and criteria according to which the processing activity takes place.
Structural adequacy refers to the interrelationships between the theoretical constructs. Whereas the physical sciences are associated with tight, logical networks of constructs, psychology is characterised by a large number of conceptual constructs with few formal connections within the nomological net. The connections, when made, tend to be descriptive and relatively loose. Where factor analytical techniques are applied to identify constructs, it should be kept in mind that factor analysis is based on matrix algebra rather than on mathematical equations and does not provide any rational equations for the linking of concepts (Royce, 1963).
Other methodological shortcomings further increase the degree to which constructs representing highly integrated processes are confounded.
Research in cognitive psychology should ideally contribute to the existing nomological network in a particular field. In other words, proposed constructs should be linked to existing constructs and these relationships investigated. With the present model this was attempted both theoretically and empirically. The interrelationships between the proposed constructs should also be specified. Whereas most existing models represent the processing constructs separately, the constructs of the present model are represented as overlapping fields of a matrix. The processes cannot be regarded as strictly hierarchically organised, nor do they subsume one another or are necessarily applied sequentially.
The proposed processing constructs are represented as overlapping fields of a matrix incorporating processing activities that are mentioned in the literature and cover major areas of task content graded according to level of complexity, as partly implied in the literature.
The more integrated the nature of processing and the higher the degree of metacognitive involvement, the more effective the processing activities are assumed to be. Because of the complicated nature of these interrelationships, correlations between the postulated constructs, revealed by means of statistical investigation, were regarded as permissible. However, it is claimed only that the postulated model has heuristic usefulness (as indicated by its construct validity) – a property regarded by Sternberg (1984) as the most important characteristic of a psychological theory given the current scientific status of the field.
The criterion of specificity refers to the definition of the processing constructs and the specifications of interrelationships. This criterion is to some extent met by the present model in that the proposed problem solving processes are described in detail and references to constructs already discussed in the literature, are made throughout.
The meta-theoretical criterion of empirical adequacy refers to the ability of a model to account for empirical findings. The results of the present study provide an indication of the model’s compliance with this criterion.
In terms of the criterion of practical utility, educational needs such as remedial, diagnostic and curriculum design purposes, as well as cognitive development in the work environment, should be considered in the construction of models of problem solving. Wagner and Sternberg (1984) noted the substantial gap between contemporary cognitive and educational psychology, and Greeno (1985), among others, emphasised the potential benefits of interdisciplinary enrichment of the two fields.
In this study an attempt was made to structure processing activities at a level both specific enough to be operationalized and general enough to cut across a broad range of problem solving activities and content domains, thereby accommodating the diversity of cognitive requirements present within the educational system.
Alongside the need for the integration of cognitive and educational psychology is the need for the consolidation of paradigms within cognitive psychology. Various attempts can be identified in this regard, such as the detailed and much publicised works of Carroll (1974) and Sternberg (1977b). Given the general lack of research findings reflecting synthesis between traditionally separate disciplines, further exploration of this area is necessary.
The proposed model represents a systems approach concerned with function as a basis for understanding structure, thereby accommodating both the process and structural approaches. This model is thus conceived at the interface between experimental and differential psychology and contributes towards the body of research emphasising the importance of increasing integration that is emerging as a trend in cognitive psychology.
Validating the CPP
The model for cognition presented in Figures 1-5 has formed the basis for the assessment tool, the CPP. The styles, attributes, and processes assessed within the CPP are derived directly from the processes defined in the model for cognition. These represent the consequences of the holonic structure and interactions between the processes identified in Figure 1. The rules for instantiation of those CPP attributes are embedded in software. To that extent they are not deployed subjectively. What is important is that the rules for instantiation of the ‘measurable/identifiable’ attributes, processes, and styles are set out as objective algorithms which produce outputs contingent upon the correct application of those rules. Validation may involve the creation of a nomological network of correlations with a cluster of other attributes, but more importantly, resides in the rules which instantiate the constructs or attributes.
Reliability
Given the scores and class/category assignments for any individual are generated via fixed computer algorithm, then, given a fixed set of inputs, the same scores and assignments will always be generated. The assessment thus has perfect reliability. So, any ‘unreliability’ is actually associated with the unique performance output of an individual on the CPP (Lumsden (1978) had already set out this proposition many years ago in connection with other kinds of questionnaire assessments). Given the assessment cannot validly be redone within a short period of time, retest reliability cannot adequately be established except perhaps over intervening periods of four years or more (to ensure that the task contents and requirements are largely forgotten). However, a number of these studies have nevertheless been undertaken. The reliability of assessment is thus solely a function of ‘person reliability’. If cognition within individuals is inherently unstable, then no assessment can yield reliable stationary estimates of attributes considered definitive of that cognition.
The Evidence-Base for the CPP
This evidence-base mainly constitutes the examination of relationships with psychometric assessments of cognitive ability, personality, and emotional intelligence assessments, as well as other assessment of learning potential. Some predictive validity studies have also been conducted relating learning styles and SSTwork level assignments to external criterion outcomes such as job-roles, work patterns and responsibilities, and academic performance. A research document summarising the available concurrent and predictive validity of CPP attributes, as well as equivalence findings and test-retest reliability results is available for download from: http://www.cognadev.com/cppResearch/The%20CPP%20Research%20Manual.pdf. There is thus a substantive body of evidence which indicates many of the CPP assignments do possess validity and utility. However, this research is ongoing, and now focusing on methods which are more sensitive to the particular measurement properties of many CPP assignments, and the non-quantitative nature of many kinds of outcomes.
Cognitive Processes and Leadership: The Implications
Cognition is a key factor in leadership considerations. A substantial body of research findings have for decades indicated the important impact of cognitive functioning on job performance. These findings largely reflect results based on traditional intelligence tests – even though compelling evidence of the weaknesses of these psychometric techniques abounds (Sarkar, 1999; Schonemann, 2005; Flynn, 2007; Gladwell, 2007; Duckworth, Quinn, Lynam, Loeber, & Stouthamer-Loeber, 2011; Richardson, 2013). The development of more effective, standardised criteria and effective tools for measuring cognitive functioning in culturally heterogeneous contexts has been emphasised as an important research goal for decades.
The need to address this measurement challenge in an innovative manner, has thus become almost mandatory given its real world implications for job performance, leadership practices and job satisfaction. Cognitive assessment results can add value to a number of HR practices such as selection and placement, team compilation, succession planning, career path planning, the identification of bursary holders, career guidance, as well as personal and team development.
The contextualisation of the CPP results in work environments and leadership settings, takes place in terms of the Stratified Systems Theory (SST) of Elliott Jaques and the Viable Systems Model (VSM) of Stafford Beer. In addition to the cognitive capability indications and the SST recommendations specified in a person’s CPP report, cognitive preferences and stylistic tendencies, also impact everyday functioning. These and other aspects of integrative personality functioning can be linked to the competency requirements of a particular position, using a job analysis system: Contextualised Competency Mapping (CCM) (Prinsloo, 2003).
Cognitive complexity and the Stratified Systems Theory (SST)
The CPP results reflect the complexity and cognitive style requirements, or the capability and preference requirements, of the various SST work environments as specified by Jaques, the VSM of Beer, and the author’s interpretation of these in terms of cognitive characteristics (Prinsloo, 2001).
The SST describes work requirements in terms of a hierarchical structure of increasing complexity and vagueness. The complexity of work, according to Jaques, is best indicated by the time frame involved. The first three levels as specified by the SST show a strong operational focus whereas the fourth and further levels are characterised by a more strategic orientation. In terms of competency requirements, each of the SST levels differs both quantitatively (increasing complexity) and qualitatively (nature of the work) from the other levels.
The CPP does not look at time frame as a criterion of work complexity, but considers stylistic preferences, work-related cognitive preferences, judgement capability and the unit of information used by the person, to calculate the complexity preferences. The manner in which CPP functioning is linked to SST requirements, is carefully explained in the CPP report. Broad guidelines in this regard are briefly summarised below:
Those best suited to the SST Pure Operational environment, are normally individuals who show less interest in intellectual complexity, vagueness and cognitive challenge. They prefer a structured context where they can experience certainty and not risk error. This may be related to the importance of other factors in their lives including interests and circumstances related to educational, interpersonal and socio-economic aspects. Those showing an operational orientation usually prefer to rely on the guidance of others and to work as a team. At times they may be expected to apply their cognitive capability in a random, inconsistent and even impulsive manner. A strong memory and intuitive approach is often indicated by their CPP profiles. They also seem to doubt their own judgement capability in complex, unfamiliar or vague environments or simply seem unaware of vague matters. Some rely on auditive modes of processing and may show well-developed verbal skills. This may particularly apply to individuals from auditive cultures but is not necessarily the case in general. Others may rely on visual modes of processing and be effective in dealing with spatial and other visual information of a tangible nature, especially where a well-developed knowledge and skills base exists. The metacognitive awareness of those plotted at a Pure Operations SST level by the CPP, usually is underdeveloped.
It is estimated that a majority of people in the work environment, almost 80%, prefer cognitive functioning in Pure Operational and Diagnostic SST environments.
Those best suited to Diagnostic work environments, can be quite analytical, but still show a need for structure – in the form of technical guidelines and/or previous experience. Although detailed, they do not necessarily follow their reasoning processes through but prefer categorising information to come to a conclusion. In a trouble-shooting role, they develop a more linear and consequential cognitive approach. They tend to accept technical assumptions in a non-critical manner and readily apply their knowledge. They enjoy variety in problem solving of a linear-causal nature and tend to investigate problems in terms of a tree-structure of either-or questions, applying rule-of-thumb principles. At this level there is a reluctance to capitalise on judgement and intuition to clarify vague issues. However, intuition is relied upon when the person is able to capitalise on previous exposure. It is interesting that those involved in practical Diagnostic work, often develop very effective metacognitive awareness to evaluate causes of problems in specific knowledge domains. Their CPP problem solving style is normally Explorative, Analytical, Reflective, Memory-based and Structured.
IQ tests measure Diagnostic capability but largely fail to access the systems thinking required at the strategic SST levels. It should also be pointed out that those with a Diagnostic orientation can be most effective in the higher educational contexts and their cognitive approach, in combination with study skills, motivation and work ethic, may enable academic achievement at the highest levels. This can be understood in terms of the relatively structured and technical nature of academic environments.
At a Tactical Strategy or Alternative Paths level of SST functioning, the capacity to extract principles that can be applied to the complex manifestations of everyday life emerges. Theoretical guidelines often need to be adapted for application. Those with a Tactical orientation no longer rely on linear processing, but prefer viewing issues in terms of tangible systems and the interaction between observable system elements. They also tend to rely more on a logical process orientation rather than on specific rules and structured information, such as in the case of the Diagnostic level. However, they do not show readiness to cope with the highly interactive and dynamic approach that is required for Parallel Processing functioning.
The Tactical strategy environments require the capacity to question assumptions, formulate hypotheses and to systematically structure unstructured information. Planning and monitoring skills become important. Curiosity and explorative tendencies may also contribute to job effectiveness. The CPP cognitive styles that are most appropriate in Tactical strategy environments, include: Structured, Logical reasoning, Analytical, Quick Insight or Intuitive, and at times, also Learning styles. At this level, intuition is still largely based on previous experience. Judgement is relied on where broad theoretical guidelines are available, and have to be applied in unfamiliar contexts.
Those showing a Tactical Strategy preference without potential to develop a Parallel Processing orientation, experience much stress in Parallel Processing environments. They also tend to reduce the job complexity by focusing on tangible and operational issues, which may impact on the long terms viability of their strategies.
Parallel Processing functioning, is associated with the capacity to accommodate novelty, vagueness, dissonance and fragmentation, all of which require the cognitive skills of integration and innovation. The level of complexity involved here, is exceedingly high and it seems that approximately only 4% of people in the corporate context feel comfortable at this level.
Parallel Processing contexts are highly changeable, dynamic and interactive. The reliance on a detailed analytical and structured cognitive approach, as required by the preceding operational levels, is replaced here by a stronger emphasis on an integrative, process approach (looking for long term implications and consequences). Logical skills in the form of both convergent and divergent reasoning are applied for transformational purposes. Individuals who prefer, and are effective in these Parallel Processing environments tend to focus on dynamic and interactive systems. They also enjoy the conceptualisation of ideas and formulation of models which support broad strategy formulation in the business context. Whereas Diagnostic thinking involves the acceptance of technical assumptions and Tactical thinking the formulation of hypotheses and plans, Parallel Processing thinking involves the critical re-evaluation and possible transformation of assumptions from which new paradigms are likely to grow. At this level new approaches are also designed and modeled. The typical CPP stylistic preferences associated with Parallel Processing functioning are: Logical reasoning, Integrative, Holistic and Quick Insight. As in Tactical contexts, learning styles may also support effectiveness here. Another prominent characteristic of the CPP profiles of individuals suited to Parallel Processing environments, is their relatively high CPP score on Judgement. This means that they “know what they don’t know”, tend to explore such vague issues optimally, clarify and prioritise fuzzy information, make decisions and contextualise these. In order to do so, they need to be in touch with their intuitive insights.
Pure Strategic functioning is characterised by a strong Intuitive and Holistic inclination. Although detail is often sacrificed in favour of the big picture, effective cognitive application involves the identification of a few detail elements by which change within the whole dynamic system can be leveraged. The unit of information used is “chaos and emerging pattern”.
The half percent of people in the corporate context whose cognitive functioning supports effectiveness in Pure Strategic environments, tend to not look for what makes sense, but show awareness of what does not make sense and store this at a subconscious levels where more complex links can be made intuitively. They also tend to transcend the detail complexity of Diagnostic and Tactical contexts and the dynamic complexity of Parallel processing environments to come up with seemingly simple intuitive solutions. This simplicity is derived at after the consideration of complex implications, though. These are, however, not merely blue sky thinkers. Effectiveness at this level requires the accommodation of complex practicalities and potential derailers. Individuals in Pure Strategic positions are often required to architecture flexible approaches around a core intent that could ensure long term systems viability.
Individuals that could effectively play leadership roles in these environments need to be able to capitalise on a broad knowledge and experience base. Cognitively they also need to accommodate emerging philosophical trends and soft, fuzzy issues related socio-political and environmental considerations. Interest in philosophical and macro-economic issues may also be characteristic of those functioning at this level.
The CPP is only linked to the first five levels of the seven level SST model. This can be explained in terms of the cognitive focus of the CPP versus the more holistic nature of the SST model. Potential for Pure Strategic functioning as indicated by the CPP is proposed to be sufficient for cognitive functioning at SST levels 6 and 7. At the highest SST levels, global exposure, leadership characteristics, as well as social and environmental awareness may be required in addition to the cognitive skills and preferences as measured by the CPP.
Cognitive style
Not only do the SST level of work indices provide useful guidance for the application of CPP results in the leadership and work contexts, but so does the issue of cognitive style. Although this construct will not be dealt with here in any depth, its practical implications will briefly be reviewed. It should be pointed out that the particular combination of stylistic preferences on the CPP provide more richness than the styles themselves. Feedback to thousands of individuals involved in different career fields, who have often also completed personality questionnaires, have shown that particular stylistic combinations are reflective of certain cognitive values and personality predispositions.
For example, Logical reasoning and Memory styles in combination often indicate a sensitive, careful, rigorous, knowledge-based approach and high personal performance standards. A combination of the Logical reasoning, Analytical and Explorative styles are typical of those who show a “left brain” orientation, such as accountants. Those preferring a Logical reasoning combined with an Integrative style show a preference for complexity, making meaning and the world of ideas. Logical reasoning combined with a Metaphoric style, indicate a strong “right brain”, ideas oriented and transformational approach such as in the case of a fair proportion of legal practitioners (but not necessarily lawyers dealing with routine, specialist applications). A purely Metaphoric style, however, may indicate a somewhat unanalytical, auditive and verbal orientation – an approach which can be very effective in negotiation. Logical reasoning combined with Learning and Quick insight indicate challenge seeking, rigour, boredom and confidence, yet self-discipline and commitment, whereas those who only show a Learning and Quick insight stylistic approach, can be expected to seek challenge and novelty, be highly adaptive and show career mobility (in certain environments, often within a two year time frame). This tendency is even more pronounced when combined with certain personality characteristics such as an MBTI: NP profile.
The effectiveness of the Memory style in particular, depends on what it is combined with. Should a Memory style be combined with a Reflective, Structured and/or Explorative approach, it indicates a focus on tangible information and a reliance on previous knowledge and experience driven by a need for certainty. It may compromise effectiveness in vague and unfamiliar contexts. Should the Memory style be combined with an Intuitive, Learning, Integrative, Holistic or Logical approach, it will further facilitate an integrative and intuitive approach.
Random and Impulsive approaches, also when in combination with an Explorative or Reflective style, are generally ineffective (but may to some extent be useful in creative environments). This tendency may be emotionally driven and be triggered by performance anxiety, which in extreme cases may render a person’s CPP results invalid. It may also be related to personality and biological factors (impulsivity, over-sensitivity, demotivation or depression) or to a history of inadequate analytical training which resulted in a lack of cognitive discipline and rigour. It can be remediated relatively easily, though – depending on factors such as age, motivation and opportunity.
Many such stylistic combinations are reported on by the CPP, all of which seem to manifest in work-related functioning too. These styles developed due to both the inherent preferences of the person as well as learning experiences. Other factors such as values, culture and consciousness may also be involved.
Contextualisation of cognitive results via Contextualised Competency Mapping (CCM)
It should be pointed out that cognitive functioning only is not sufficient to predict something as complex as work performance. A more holistic picture of integrated psychological functioning is required to optimise person-job matching. Cognitive assessment results can, however, be regarded as valuable prerequisites for performance, especially in complex work domains. Cognitive results tend to predict failure, but do not guarantee performance. The holistic assessment of cognition in conjunction with values and motivational factors is thus recommended.
The Contextualised Competency Mapping (CCM) technique has been developed to contextualise psychometric results by linking it to the competency requirements of a particular job. The CCM analyses the cognitive and the holistic (personality, values, motivation, emotional intelligence) requirements of work plus contains a 360 degree evaluation functionality. A person’s test results (CPP, LOI, VO, MP, 360 degree) can also automatically be linked to the job requirements. A CCM report is automatically generated to graphically indicate and narratively explain the degree of person-job matching. This report is used in the work environment for purposes of selection and placement, personal and team development and career pathing or succession planning.
Conclusion
Cognitive skill is an important prerequisite for effective leadership, which in turn can be regarded as the proprietor of social and environmental sustainability. The CPP provides an example of a dynamic modelling technique aimed at addressing the issue of cognitive performance in the leadership and work contexts in an innovative and practically useful way. The approach presented here may stimulate new thinking on cognition and its implications for leadership. It can be predicted that the current emphasis on “ability” in intelligence research is bound to gradually make way for a focus on “cognitive processes”, which in turn may be overtaken by increased interest in the understanding of “consciousness” and associated concepts such as wisdom, intuition, integrity and intent, given the potentially critical impact of these concepts within the domain of leadership. Although mere speculation, such research developments may offer more than current practice which provides few guarantees in identifying and developing leaders at all levels of society to address, with consciousness and compassion, complex macro-system challenges. In alignment with Geertz’s (1973) view of the goal of research as discovering a “content of meaning”, the theoretical model and measurement techniques proposed in this paper are aimed at making a contribution of a heuristically and scientifically useful scope within the important field of human thought.
References
Anderson, J. R. (1975). Cognitive Psychology: The study of knowing, learning and thinking. New York: Academic Press.
Anderson, J. R. (1985). Cognitive Psychology and its applications (2nd ed.). New York: W H Freeman and Company.
Bartholomew, D.J., Deary, I.J., & Lawn, M. (2009). A new lease of life for Thomson’s bonds model of intelligence. Psychological Review, 116, 3, 567-579.
Baron, J. B. (1982). Personality and Intelligence. In R. J. Sternberg (Ed.), Handbook of human intelligence. Cambridge, Massachussetts: Cambridge University Press.
Baron, J. B. (1984). Criteria and explanations. The Behavioural and Brain Sciences, 2, 2, 287-288.
Berry, J. W. (1974). Psychological aspects of cultural pluralism: Unity and identity reconsidered. Topics in Cultural Learning, 2, 239-252.
Berry, J. (1984). Multicultural policy in Canada: A social psychological analysis. Canadian Journal of Behavioural Sciences, 16, 353-370.
Binet, A., & Simon, T. (1980). The development of intelligence in children (trans. E.S. Kite, with L.M. Terman’s marginal notes and L. M.Dunn(ed.)). Nashville: Williams Printing.
Bogen, J., & Woodward, J. (1988). Saving the phenomena. Philosophical Review, 97, 303–352.
Broughton, J.M. (1981). Piaget’s structural developmental psychology: V. Ideology-critique and the possibility of a critical developmental theory. Human Development, 24, 6, 382-411.
Burt, C. (1949). The structure of the mind: A review of the results of factor analysis. British Journal of Educational Psychology, 19,100-114 & 176-199.
Camazine, S., Deneubourg, J-L., Franks, N.R., Sneyd, J., Theraulaz, G., & Bonabeau, E. (2001). Self-Organization in biological systems. New Jersey: Princeton University Press.
Carroll, J. B. (1974). Psychometric tests as cognitive tasks: A new “Structure of IntellectÓ (Technical Report No. RB-74-16). Princeton, NJ: Educational Testing Service.
Carroll, J. B. (1981). Ability and task difficulty in cognitive psychology. Educational Researcher, 10, 11-21.
Carroll, J. B., & Maxwell, S. E. (1979). Individual differences in cognitive abilities. Annual Review of Psychology, 30, 603-640.
Castellanos, N.P., Leyva, I., Buld˙, J.M., Bajo, R., Pa˙l, N., Cuesta, P., Ord€“ez, V.E., Pascua, C.L., Boccaletti, S., Maest˙, F., & del-Pozo, F. (2011). Principles of recovery from traumatic brain injury: Reorganization of functional networks. Neuroimage, 55, 3, 1189-1199.
Castles, E. E. (2012). Inventing Intelligence: How America Came to Worship IQ. Santa Barbara, CA: Praeger/ABC-CLIO.
Chi, M. T. H., Feltovich, P. J., & Glaser, R. (1985). Categorization and representation of physics problems by experts and novices. Cognitive Science, 5, 121-152.
Chipman, S. F., Segal, J. W., & Glaser, R. (Eds.). (1985). Thinking and learning skills (Vol. 2). Hillsdale, NJ: Lawrence Erlbaum.
Colberg, M., Nester, M. A., & Trattner, M. H. (1985). Convergence of the inductive and deductive models in the measurement of reasoning abilities. Journal of Applied Psychology, 70, 4, 681-694.
Craik, F. I., & Lockhart, R. S. (1972). Levels of processing: A framework for memory research. Journal of Verbal Learning and Verbal Behaviour, 11, 671-684.
Cronen, V. E., & Michevc, N. (1972). The evaluation of deductive argument: A process analysis. Speech Monographs, 39 (2), 124-131.
Dellarosa, D. (1988). A history of thinking. In R.J. Sternberg & E.E. Smith (Eds.), The Psychology of human thought (Chapter1, pp. 1-18). New York: Cambridge University Press.
Detterman, D. K. (1984). Understanding cognitive components before postulating metacomponents. part 2. The Behavioural and Brain Sciences, 2,2, 289-290.
Duckworth, A.L., Quinn, P.D., Lynam, D.R., Loeber, R., & Stouthamer-Loeber, M. (2011). Role of test motivation in intelligence testing. PNAS, 108, 19, 7716-7720.
Economos, J. (1984). Intelligent dissention among the Archoi is good for the people. The Behavioural and Brain Sciences, 1,2, 290.
Edelmann, J.B., & Denton, M.J. (2007). The uniqueness of biological self-organization: challenging the Darwinian paradigm. Biology and Philosophy, 22, 4, 579-601.
Egan, D. E., & Gomez, L. M. (1985). Assaying, isolating, and accommodating individual differences in learning a complex skill. In R. F. Dillon (Ed.), Individual Differences in Cognition (pp. 173-217). Orlando: Academic Press.
Eliasmith, C., Stewart, T.C., Choo, X., Bekolay, T., DeWolf, T., Tang, C., & Rasmussen, D. (2012). A large-scale model of the functioning brain. Science, 338, 6111, 1202-1205.
Ellis, N. R., Meador, D. M., & Bodfisch, J. W. (1985). Differences in intelligence and automatic memory processes. Intelligence, 9, 265-273.
Embretson, S. E., Schneider, L. M., & Roth, D. L. (1986). Multiple processing strategies and the construct validity of verbal reasoning tests. Journal of Educational Measurement, 23(1), 13-32.
Erickson, J. R. (1974). A set analysis theory of behavior in formal syllogistic reasoning tasks. In R. Solso (Ed.), Loyola symposium on cognition (Vol. 2, pp. 305–330). Hillsdale, NJ: Erlbaum.
Ertl, J. P., Schafer, E. W. P. (1969). Brain response correlates of psychometric intelligence. Nature, 223, 421-422.
Estes, W. K. (1986). Memory storage and retrieval processes in category learning. Journal of Experimental Psychology: General, 115(2), 155-174.
Evans, J. St B. T. (1982). The Psychology of deductive reasoning. London: Routledge & Kegan Paul.
Eysenck, H. J. (1984). Intelligence versus behaviour. The Behavioural and Brain Sciences, 1, 2, 290-291.
Eysenck, H. J. (1986). The theory of intelligence and the psychophysiology of cognition. In R. J. Sternberg (Ed.), Advances in the Psychology of human intelligence (Vol. 3, pp. 1-34). Hillsdale, NJ: Lawrence Erlbaum.
Feldhusen, J. F., Houtz, J. C., & Ringenbach, S. (1972). The Purdue Elementary Problem-solving Inventory. Psychological Reports, 91, 891-901.
Flynn, J.R. (2007). What is Intelligence? New York: Cambridge University Press.
Ford, M.E., & Tisak, M.S. (1983). A further search for social intelligence. Journal of Educational Psychology, 75, 196-206.
Frederiksen, C. H. (1986). Cognitive models and discourse analysis. In C. R. Cooper 8: S. Greenbaum (Eds.), Written communication annual, Vol. I: Studying writing: Linguistic approaches (pp. 227-267). Beverly Hills, CA: Sage.
Feuerstein, R., Rand, Y., Hoffman, M. B., & Miller, R. (1980). Instrumental enrichment: An intervention programme for cognitive modifiability. Baltimore: University Park Press.
Gallagher, S. & Reid, D.K. (1981). The learning theory of Piaget and Inhelder. Monterey, CA: Brooks/Cole.
Galton, F. (1883). Inquiries into human faculty and its development. London: J.M. Dent & Co.
Garlick, D. (2002). Understanding the Nature of the General Factor of Intelligence: The Role of Individual Differences in Neural Plasticity as an Explanatory Mechanism. Psychological Review, 109, 1, 116-136.
Geman, S. (1981). Notes on a self-organizing machine. In G. E. Hinton & J. A. Anderson (Eds.), Parallel models of associative memory (pp. 237-264). Hillsdale, NJ: Lawrence Erlbaum.
Geertz, J. C. (1973), Thick description: Towards an interpretive theory of culture. In C. Geertz, The interpretation of cultures: selected essays, pp 3 – 30. New York: Basic Books.
Gitomer, D. H., & Pellegrino, J. W. (1985). Developmental and individual differences in long term memory retrieval. In R. F. Dillon (Ed.), Individual differences in cognition (Vol. 2, pp. 1-34). Orlando: Academic Press, Inc.
Gladwell, M. (2007). None of the above: what IQ doesn’t tell you about race. New Yorker magazine, Dec 17th, 92-96. Retrieved from: http://www.newyorker.com/arts/critics/books/2007/12/17/071217crbo_books_gladwell?printable=true
Goldsmith, R.E., & Matherly, T.E. (1986). Seeking simpler solutions: Assimilators and explorers, adaptors and innovators. The Journal of psychology, 120, 2, 149-155.
Gordon, E. W., & Terrell, M.D. (1981). The changed social context of testing. American Psychologist, 36, 10, 1167.
Gottfredson, L. S. (1997). Mainstream science on intelligence: An editorial with 52 signatories, history, and bibliography. Intelligence, 24(1), 13–23.
Greeno, J. G. (1985). Looking across the river: Views from the two banks of research and development in problem solving. In S. F. Chipman, J. W. Segal & R. Glaser (Eds.), Thinking and learning skills (Vol. 2, pp. 209-213). Hillsdale, NJ: Lawrence Erlbaum.
Guilford, J. P. (1967). The nature of human intelligence. New York: McGraw Hill.
Gur, R.C., Turetsky, B.I., Matsui, M., Yan, M., Bilker, W., Hughett, P., & Gur, R.E. (1999). Sex Differences in Brain Gray and White Matter in Healthy Young Adults: Correlations with Cognitive Performance. The Journal of Neuroscience, 19, 10, 4065-4072.
Gustafsson, J. E. (1988). Hierarchical models of individual differences in cognitive abilities. In R. J. Sternberg (Ed.), Advances in the psychology of human intelligence, Vol. 4 (pp.35–71). Hillsdale, N.J.: Lawrence Erlbaum Associates, Inc.
Guttman, L. (1965). A facetted definition of intelligence. Scripta Hierosolymitana, 14,161-181.
Haig, B. (2005). An abductive theory of scientific method. Psychological Methods, 10, 4, 371-388.
Haig, B. (2013) Detecting psychological phenomena: Taking bottom-up research seriously. American Journal of Psychology, 126, 2, 135-153.
Hendrickson, D.E., & Hendrickson, A.E. (1980). The biological basis of individual differences in intelligence. Personality and Individual Differences, 1, 3-33.
Henle, M. (1962). On the relation between logic and thinking. Psychological Review, 6341, 366-378.
Hirschman, E. C. (1981). Some novel propositions concerning problem solving. Perceptual and Motor Skills, 52, 523-536.
Horn, J.L. (1970). Organization of Data on Life-Span Development of Human Abilities. In L. R. Goulet and P. B. Baltes (Eds.), Life-Span Developmental Psychology: Research and Theory (pp. 423-466). New York: Academic Press.
Horn, J.L. (1978). Human ability systems. Life-span Development and Behavior, 1, 211-256.
Horn, J.L. (1986). Intellectual ability concepts. In R. J. Sternberg (Ed.), Advances in the Psychology of human intelligence, 3, 35-77. Hillsdale, NJ: Lawrence Erlbaum.
Howe, M. J. A. (1988). Intelligence as an explanation. British Journal of Psychology, 79, 3, 349-360.
Hunt, E., & Lansman, M. (1986). Unified model of attention and problem solving. Psychological Review, 133(4), 446-461.
Hurlbert, A., & Poggio, T. (1985). Spotlight on attention. Trends in Neurosciences, 8, 309-311.
Jackson, N. E. (1984). Intellectual giftedness: A theory worth doing well. The Behavioural and Brain Sciences, 1, 2, 294-295.
Jensen, A. R. (1998). The g factor. Westport, CT: Praeger.
Keating, D. (1984). The emperor’s new clothes: The new look in intelligence research. In R. J. Sternberg (Ed.), Advances in the Psychology of human intelliqence (Vol. 2, pp. 1-45). Hillsdale, NJ: Lawrence Erlbaum.
Knaeuper, A., & Rouse, W. B. (1985). A rule-based model of human problem solving behaviour in dynamic environments. Institute of Electrical and Electronics Engineers Transactions on Systems, Man and Cybernetics, 15, 6, 708-719.
Kohlers, P. A. (1979). A pattern analyzing basis of recognition. In L. S. Cermak & F. I. M. Craik (Eds.), Levels of processing in human memory (pp. 363-384). Hillsdale, NJ: Lawrence Erlbaum.
Larkin, J. H. (1985). Understanding problem representation and skill in physics. In S. F. Chipman, J. E. Segal & R. Glaser (Eds.), Thinking and learning skills (Vol. 2, 141-159). Hillsdale, NJ: Lawrence Erlbaum.
Larson, G. E., & Sacusso, D. P. (1989). Cognitive correlates of general intelligence: Towards a process theory of g. Journal of experimental Psychology: Learning, Memory and Cognition, 1, 5-31.
Lumsden, J. (1978) Tests are perfectly reliable. British Journal of Mathematical and Statistical Psychology, 31, 1, 19-26.
Maraun, M.D. (1998). Measurement as a Normative Practice: Implications of Wittgenstein’s Philosophy for Measurement in Psychology. Theory & Psychology, 8, 4, 435-461.
Meichenbaum, D. (1980). A cognitive behavioural perspective on intelligence. Intelligence, 4, (4), 271-283.
Michell, J. (2009). The psychometricians’ fallacy: Too clever by half? British Journal of Mathematical and Statistical Psychology, 62, 1, 41-55.
Michell, J. (2012). Alfred Binet and the concept of heterogeneous orders. Retrieved from:
http://www.frontiersin.org/quantitative_psychology_and_measurement/10.3389/fpsyg.2012.00261/abstract Frontiers in Quantitative Psychology and Measurement, 3, 261, 1-8.
Michell, J. (2013). Constructs, inferences, and mental measurement. New Ideas in Psychology, 31, 1, 13-21.
Miller, E. M. (1994). Intelligence and brain myelination: A hypothesis. Personality and Individual Differences, 17, 6, 803-832.
Minsky, M. (1974). A framework for representing knowledge. Springfield: National Technical lnformation Services, US Department of Commerce.
Neisser, U. (1967). Cognitive Psychology. New York: Appleton Century Crofts.
Neisser, U., Boodoo, G., Bouchard, T., J., Boykin, A.W., Brody, N., Ceci, S.J., Halpern, D.F., Loehlin, J.C., Perloff, R., Sternberg, R., & Urbina, S. (1996). Intelligence: Knowns and unknowns. American Psychologist, 51, 2, 77-101.
Newell, A. (1973). Production systems: Models of control structures. In W. G. Chase (Ed.), Visual information Processing (pp. 463-526). New York: Academic Press.
Newell, A., & Simon, H. A. (1972). Human problem solving. Englewood Cliffs, NJ: Prentice-Hall.
Nisbett, R.E., Aronson, J., Blair, C., Dickens, W., Flynn, J., Halpern, D.F., & Turkheimer, E. (2012). Intelligence: New findings and theoretical developments. American Psychologist, 67, 2, 130-159.
Peirce, C. S. (1931–1958). Collected papers (Vols. 1–8),C. Hartshorne, P. Weiss, & A. Burks, Eds.). Cambridge, MA: Harvard University Press.
Pellegrino, J. W., & Glaser, R. (1979) Cognitive correlates and components in the analysis of individual differences. Intelligence, 3, 187-214.
Poole, D., Mackworth, A., & Goebel, R. (1998). Computational intelligence: A logical approach. New York: Oxford University Press.
Popper, K. (1959). The logic of scientific discovery. Hutchinson, London.
Pribram, Karl H. (1986). The cognitive revolution and mind-brain issues. American Psychologist, 41, 5, 507-520.
Prinsloo, M. (1992). A theoretical model and empirical technique for the study of problem solving processes. PhD. RAU, Johannesburg.
Prinsloo, M., & Prinsloo, R. (2001). The Cognitive Process Profile (CPP) user’s manual. Johannesburg: Cognadev.
Prinsloo, M. (2003). The Contextualised Competency Mapping (CCM) user’s manual. Johannesburg: Cognadev.
Prinsloo, M. (2012). Consciousness models in action: comparisons. Integral leadership Review, June, Retrieved from: http://integralleadershipreview.com/7166-consciousness-models-in-action
Ratcliff, R. (1981). A theory of order relations in perceptual matching. Psychological Review, 88, 552–572.
Raz, N., Millman, D., & Sarpel, G. (1990). Cerebral correlates of cognitive aging: Gray-white-matter differentiation in the medial temporal lobes, and fluid versus crystallized abilities. Psychobiology, 18, 4, 475-481.
Resnick, L. B., & Glaser, R. (1976). (1976). Problem solving intelligence. In Resnick L. (Ed.). The nature of intelligence (pp. 205–230). New York: John Wiley & Sons
Richardson, K. (2013). The eclipse of heritability and the foundations of intelligence. New Ideas in Psychology, 31, 2, 122-129.
Rips, L.J., (1994). The Psychology of Proof: Deductive Reasoning in Human Thinking. The MIT Press, Cambridge, MA.
Roy, A. (2012). A theory of the brain: Localist representation is used widely in the brain. Frontiers in Cognitive Science, 3, Dec, 1-4.
Royce, J. R. (1963). Factors as theoretical constructs. American Psychologist, 18, 522-528.
Royce, J. R., & Powell, A. (1983). Theory of Personality and Individual differences: Factors, systems and processes. Englewood-Cliffs, NJ: Prentice-Hall, Inc.
Rumelhart, D. E., & Ortony, A. (1977). The representation of knowledge in memory. In R. C. Anderson, R. de J. Spiro & W. E. Montague (Eds.), Schooling and the acquisition of knowledge (pp. 99-135). Hillsdale, NJ: Lawrence Erlbaum.
Sarkar, S. (1999). Delusions about IQ. Current Psychology of Cognition, 18, 2, 224-231.
Schank, R. C., & Childers, P. G. (1984). Understanding and intelligence. In R. C. Schank & P. G. Childers (Eds.), The cognitive computer: On language learning and artificial intelligence (pp. 110-133). Massachussetts: Addison Wesley Publishing Company.
Schlechter, T.M., & Toglia, M.P. (Eds.) (1985). New directions in cognitive science. Norwood, N.J: Ablex Publishing Corporation.
Schonemann, P. (2005). Psychometrics of Intelligence. In K. Kemp-Leonard. (Eds). Encyclopedia of Social Measurement, pp. 193-201. New York: Elsevier.
Schultz, K., & Lochhead, J. (1988). Toward a unified theory of problem solvinq: A view from physics. Paper presented at the annual meeting of the American Educational Research Association, New Orleans, LA.
Schneider, W. (1985). Developmental trends in the meta-memory behaviour relationship: An integrative review. In D. L. Forrest-Pressley, G. T. McKinnon & T. G. Waller (Eds.), Metacognition, cognition and human performance, vol 1 (pp. 57-109). New York: Academic Press.
Scribner, S. (1986). Thinking in action: some characteristics of practical thought. In R.J. Sternberg & R. K. Wagner (Eds.), Practical Intelligence: Nature and Origins of Competence in the Everyday World, Chapter 2 (pp. 13-30). New York: University of Cambridge Press.
Siegler, R. S. (1981) Developmental sequences within and between concepts. Monographs of the Society for Research in Child Development, 46, (Whole No. 189).
Sigel, I.E., & Cocking, R.R. (1977). Cognitive development from childhood to adolescence: A constructivist perspective. New York: Holt, Rinehart and Winston.
Simon, H.A. (1979). Rational Decision Making in Business Organizations. American Economic Review, 69, 4,493-513.
Simon, H. A., & Kotovsky, B. (1963). Human acquisition of concepts for sequential patterns. Psychological Review, 70, 534-546.
Snow, R. E. (1979). Theory and method for research on aptitude processes. In R. J. Sternberg & D. K. Detterman (Eds.), Human intelligence (pp. 105-137). Norwood, NJ: Ablex.
Snow, R.E., Kyllonen, P.C., & Marshalek, B. (1984). The topography of ability and learning correlations. In R. J. Sternberg (ed.), Advances in the psychology of human intelligence, Vol. 2 (pp. 47-103). London: Lawrence Erlbaum.
Spearman, C. (1923). The nature of intelligence and the principles of cognition. London: MacMillan.
Spiro, R. J. (1977). Remembering information from text: The “state of schema” approach. In J. A. Anderson, R. J. Spiro & W. E. Montague (Eds.), Schooling and the acquisition of knowledge (pp. 137-177). Hillsdale, NJ: Lawrence Erlbaum.
Stehle, P. (1994) Order, Chaos, Order: The Transition from Classical to Quantum Physics. New York: Oxford University Press.
Sternberg, R. J. (1977a). The information processing approach to intelligence. In R. J. Sternberg (Ed.). Intelligence, information processing and analogical reasoning (pp. 37-64). Hillsdale, NJ: Lawrence Erlbaum.
Sternberg, R. J. (1977b). Intelligence, information processing and analogical reasoning. Hillsdale, NJ: Lawrence Erlbaum.
Sternberg, R. J. (1977c). Component processes in analogical reasoning. Psychological Review, 84, 4, 353-378.
Sternberg, R. J. (1979). A review of “Six authors in search of a character”: A play about intelligence tests in the year 2000. In R. J. Sternberg & D. K. Detterman (Eds.), Human Intelligence (pp. 257-269). Norwood, NJ: Ablex.
Sternberg, R. J. (1981). The evolution of theories of intelligence. Intelligence, 5, 209-230.
Sternberg, R. J. (I983). Components of human intelligence. Cognition, 15, 1-48.
Sternberg, R. J. (1984). A contextualist view of the nature of intelligence. International Journal of Psychology, 19, 309-334.
Sternberg, R. J. (1985). Beyond IQ: A triarchic theory of human intelligence. New York: Cambridge University Press.
Sternberg, R. J. (1986). Toward a unified theory of human reasoning. Intelligence, I0, 4, 281-314.
Sternberg, R. J. (1988). Intelligence. In R. J. Sternberg & E. E. Smith (Eds.), The psychology of human thought (pp. 267-308). Cambridge: Cambridge University Press.
Sternberg, R. J., & Detterman, D.K. (eds.) (1986). What is Intelligence? Contemporary viewpoints on its nature and definition. New Jersey: Ablex Publishing Corporation.
Sternberg, R. J., & Rifkin, B. (1979). The development of analogical reasoning processes. Journal of Experimental and Child Psychology, 27, 195-232.
Sternberg, R. J., & Smith, E. E. (Eds.). (1986). The psychology of human thought. Cambridge: Cambridge University Press.
Sternberg, R. J., & Spear, L. C. (1985). The triarchic theory of mental retardation. International Review of Research in Mental Retardation, l3, 301-326.
Strayer, D. L., & Kramer, A. F. (1990). Attentional requirements of automatic and controlled processing. Journal of Experimental Psychology: Learning, Memory and Cognition, 16, 7,-82.
Sweller, John (1988). Cognitive load during problem solving: Effects on learning. Cognitive Science, l2, 257-285.
Ter Hark, M. (1990). Beyond the inner and the outer. Dordrecht: Kluwer Academic Publishers.
Toga, A.W., Clark, K.A., Thompson, P.M., Shattuck, D.W., & Van Horn, J.D. (2012). Mapping the human connectome. Neurosurgery, 71, 1, 1-5.
Thomson, G. (1916). A hierarchy without a general factor. British Journal of Psychology, 8, 3, 271-281.
Treisman, A. (1979). The psychological reality of levels of processing. In L. S. Cermak & F. I. M. Craik (Eds.), Levels of processing in human memory (pp. 301-330). Hillsdale, NJ: Lawrence Erlbaum.
Van Der Maas, H.L., Dolan, C.V., Grasman, R.P.P., Wicherts, J.M., Huizenga, H.M., & Raijmakers, M.E.J. (2006). A Dynamical Model of General Intelligence: The Positive Manifold of Intelligence by Mutualism. Psychological Review, 113, 4, 842-861.
Van der Veer, R., & Van Ijzendoorn, M. H. (1985). Vygotsky’s theory of the higher psychological processes: Some criticisms. Human Development, 28, 1-9.
Verster, John M. (1982). A cross-cultural study of cognitive processes using computerized tests. Unpublished doctoral dissertation, UNISA, Pretoria.
Vogel, W., & Broverman, D.M. (1964). Relationship between EEG and test intelligence: A critical review. Psychological Bulletin, 62, 2, 132-144.
Vygotsky, Lev S. (1978). Mind in society. The development of higher psychological processes. Cambridge: Harvard University Press. Cambridge, Massachusetts.
Wagner, R.K. (1987). Tacit knowledge in everyday intelligent behavior. Journal of Personality and Socia1 Psychology, 52, 1236-1247.
Wagner, W. K., & Sternberg, R. J. (1984). Alternative conceptions of intelligence and their implications for education. Review of Educational Research, 54, 2, 79-223.
Wardell, D. M., & Royce, J. R. (1978). Toward a multi-factor theory of styles and their relationships to cognition and affect. Journal of Personality, 46(3), 474-505.
Wason, P. C., & Johnson-Laird, P. N. (1972). Psychology of reasoning: Structure and content (Vol. 86). Harvard University Press.
Wedeen, V.J., Rosene, D.L., Wang, R., Dai, G., Mortazavi, F., Hagmann, P., Kaas, J.H., & Tseng, W.I. (2012). The geometric structure of the brain fiber pathways. Science, 335, 6076, 1628-1634.
Whimbey, A., & Lochhead, J. (1980). Problem solving and comprehension: A short course in analytical reasoning (2nd ed.). Philadelphia: Franklin Institute Press.
Whiteley, S. E. (1973). Types of relationships in reasoning by analogy. Dissertation Abstracts International, 34 (S-B), 2292-B.
Whiteley, S.E. (1977). Information processing on intelligence test items: Some response components. Applied Psychological Measurement, 1, 465-476.
Whiteley, S. E., & Barnes, G. M. (1979). The implications of processing event sequences for theories of analogical reasoning. Memory and Cognition, 2, 4, 232-331.
Wilber, Ken (2000). Integral psychology. Boston, MA: Shambhala.
Wilber, K. (2005) Toward a comprehensive theory of subtle energies. Explore, 1, 4, 252-270.
Woodward, J. (1989). Data and phenomena. Synthese, 79, 393– 472.
Woodward, J. (2000). Data, phenomena, and reliability. Philosophy of Science, 67(Suppl.), 163–179.
About the Authors
Maretha Prinsloo is a registered psychologist who has worked extensively in the fields of Psychotherapy, Psychometric test development, People assessment and development, Project management, Research and Development, Organisational Transformation and Community Development. She worked in the fields of clinical and counseling psychotherapy from 1984 -1988 after which she turned her attention to research on personality and cognitive assessment. Completing her doctorate in Cognitive Psychology in 1992 (titled: “A theoretical model and empirical technique for the study of problem solving processes”) she went on to found the company Magellan Consulting (pty) Ltd. which she has lead since 1994. The business is currently serving approximately 1000 corporate clients and supports many independent consulting groups in the fields of people assessment and development. Magellan has expanded to the UK where it is registered as Cognadev UK Ltd and where it operates in association with a number of consulting groups that provide training, support as well as assessment and development products to clients globally.
Projects that Maretha is currently involved in include: support of organisational transformation initiatives; identification of bursary candidates; succession planning in leadership; selection and placement; executive coaching; compiling strategic teams; career guidance; development of strategic thinking; development of analytical skills; development of EQ; competency assessment; cross-cultural research; development of assessment products and managing and marketing her business. Clients include corporates representing most industries as well as independent consultants. Maretha is particularly interested in research and development which involves project management, programming, and the provision of assistance to Masters and Doctoral students. She has developed several assessment and development products.
Paul Barrett received his Ph.D. in personality psychometrics from the University of Exeter, UK. He was a research scientist and eventually co-director of the Biosignal Lab at the University of London’s Institute of Psychiatry for 14 years, Chief Scientist at two of the UK’s High Security Forensic Psychiatric hospitals, Chief Psychologist at Mariner7 (Carter Holt Harvey plc, NZ), Chief Research Scientist at Hogan Assessment Systems Inc (US), and adjunct Professor of Psychometrics within the University of Auckland Business School, NZ. Currently he is Chief Research Scientist at Cognadev/Magellan (UK and SA), an Honorary Professor of Psychology at the University of Auckland (NZ) and adjunct Professor of Psychology at the University of Canterbury (NZ). He is an author of several commercial psychological test instruments; developer of the graphical profiler class of psychological assessment technologies, and an author of over 120 research articles and book chapters. He is an associate editor of the academic journal Personality and Individual Differences, a member of the editorial board of the journal Evolutionary Psychology, a consulting editor for the Journal of Personality Assessment, Journal of Experimental Education, Frontiers in Quantitative Psychology and Measurement, and a consulting reader for the journals Perceptual and Motor Skills and Psychological Reports. He is a UK BPS Chartered Psychologist (Associate Fellow) and UK Chartered Scientist. His fields of expertise span measurement theory and psychometrics, validity and commercial evidence-base construction and evaluation, the psychology and assessment of individual differences, and non-quantitative computer-intensive analysis methodologies.